How to Become an Ai Entrepreneur
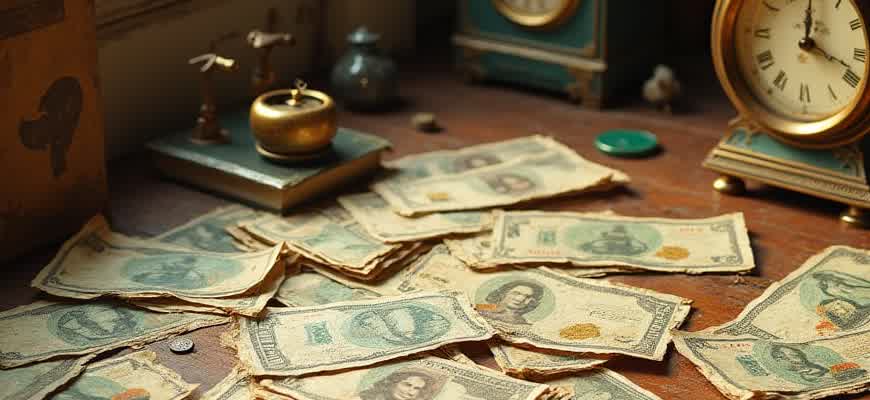
Starting a business in the artificial intelligence sector requires a strategic approach, from mastering the fundamentals to scaling innovative solutions. Here’s how you can navigate the journey:
- Learn the Fundamentals of AI: Understanding machine learning, neural networks, and data analysis is crucial. Consider enrolling in specialized courses or attending workshops to gain a solid foundation.
- Identify Market Opportunities: Analyze industries where AI can bring value. From healthcare to finance, there are countless areas where AI can create new efficiencies.
- Build a Network: Connect with AI experts, potential clients, and investors. Join communities, attend conferences, and actively engage on platforms like LinkedIn and GitHub.
Once you’ve acquired the basics, the next phase is ideation and development.
Focus on solving real-world problems. AI products that meet market needs are far more likely to succeed than experimental technologies.
Consider creating a minimum viable product (MVP) to test your ideas quickly. Start small, but make sure your product offers a unique value proposition.
- Develop a prototype that addresses a specific problem in your target market.
- Use customer feedback to refine and iterate on your solution.
- Secure early-stage funding to support further development and marketing efforts.
As you move forward, it's important to focus on scaling and acquiring users.
Phase | Key Actions | Outcomes |
---|---|---|
Prototype | Build MVP, collect feedback | Understand market fit, refine product |
Scale | Expand team, develop marketing strategy | Grow user base, increase revenue |
How to Build a Successful AI Startup
Starting an AI-driven business involves understanding the technical, business, and market aspects of artificial intelligence. As an aspiring AI entrepreneur, your primary focus should be on finding a problem that can be solved through AI, building a reliable solution, and creating a business model around it. You will need a deep understanding of AI technologies, but just as important is knowing how to leverage them to create a unique product or service that adds value to your target audience.
The path to becoming an AI entrepreneur is not linear and requires a combination of technical expertise, business acumen, and a willingness to innovate. Successful entrepreneurs in AI often start by identifying niches in various industries such as healthcare, finance, logistics, or customer service, where AI solutions can lead to increased efficiency or improved outcomes.
Steps to Launch Your AI Business
- Identify a Market Need - Before diving into technical development, ensure that there is a clear problem that AI can solve in a particular market. Start with thorough research to understand the pain points of potential customers.
- Acquire Technical Skills or Build a Team - While you don't have to be a technical expert, understanding AI fundamentals is crucial. If you're not technically inclined, consider partnering with or hiring AI specialists to bring your vision to life.
- Develop a Prototype - Once you have a solid understanding of the problem and technical approach, begin developing a prototype or a minimum viable product (MVP) to validate your ideas.
- Test and Iterate - Continually refine your solution based on user feedback and market response. Iteration is key to adapting your product to meet customer needs effectively.
- Create a Scalable Business Model - The ability to scale is essential. Determine how your product or service can grow, whether through partnerships, expanding into new markets, or improving existing capabilities.
Success in AI entrepreneurship relies heavily on finding a balance between innovative technology and the practical needs of the market.
Essential Tools and Technologies
Technology | Use Case |
---|---|
Machine Learning Algorithms | Develop predictive models for data-driven decision making. |
Natural Language Processing | Power chatbots, language translation, sentiment analysis. |
Computer Vision | Facial recognition, object detection, autonomous systems. |
Key Considerations for AI Entrepreneurs
- Ethical Implications - AI solutions should be developed with a focus on transparency, fairness, and accountability.
- Data Privacy - Ensure that any data used in your AI models is secure and follows regulatory guidelines like GDPR.
- Access to Capital - AI ventures often require significant upfront investment for research, development, and talent acquisition. Consider seeking funding from venture capitalists or AI-specific grants.
Identifying Market Gaps for AI Solutions
One of the first steps in becoming an AI entrepreneur is identifying market gaps where AI can provide significant value. These gaps are often areas where traditional solutions fail to address key pain points or where automation can improve efficiency. Recognizing these opportunities requires a deep understanding of existing industries, emerging technologies, and the specific needs of target audiences.
AI entrepreneurs should focus on finding areas where existing software solutions are limited, or manual processes are still prevalent. It is essential to analyze sectors with high growth potential and areas where AI can create a substantial competitive advantage. By identifying underserved niches, AI entrepreneurs can target solutions with clear value propositions that address real-world problems.
Approaches to Identifying Market Gaps
- Conducting thorough market research
- Engaging in conversations with industry experts and potential users
- Analyzing current technological trends and gaps in AI applications
- Identifying inefficiencies in existing processes that AI could streamline
Important Insight: Effective market gap identification goes beyond just finding problems; it's about understanding how AI can solve them better than current solutions.
Steps for Finding Gaps
- Research existing industries to understand the most significant pain points.
- Evaluate current AI applications and their limitations.
- Survey potential customers to gather insights on their needs.
- Test and validate ideas through small-scale pilots or MVPs.
Examples of Market Gaps in AI
Industry | Gap | Potential AI Solution |
---|---|---|
Healthcare | Manual diagnosis and inefficient patient record management | AI-powered diagnostic tools and electronic health record automation |
Finance | Slow and error-prone manual transaction processing | AI for fraud detection and automated transaction analysis |
Retail | Lack of personalized customer experiences | AI-driven recommendation engines and personalized shopping assistants |
Building a Scalable AI Product from Scratch
Creating a scalable AI product requires a strategic approach to both technology and business development. The process involves defining clear use cases, identifying the right data sources, and selecting appropriate algorithms that can grow with the business. An AI solution must be flexible enough to handle increasing complexity and data volume as the product scales. Below are key steps to consider when building such a product.
The foundation of a scalable AI product is its ability to adapt and evolve. A well-architected system will allow for seamless integration of new features, scalability to handle growing data, and robustness to function in real-world environments. Each decision made in the development phase plays a role in the long-term success and sustainability of the product.
Key Steps to Build a Scalable AI Product
- Define the Problem Clearly: Before jumping into the technical details, outline a specific problem that the AI product aims to solve. This clarity will guide algorithm selection and data collection efforts.
- Focus on Data Quality: AI models are only as good as the data they are trained on. Gathering high-quality, relevant data is essential to train models that perform well in the long run.
- Choose the Right Algorithms: Different tasks require different algorithms. Carefully consider whether supervised learning, unsupervised learning, or reinforcement learning is best suited for your use case.
- Ensure Scalability: Plan infrastructure and model deployment to support growth. Leverage cloud technologies and distributed systems for flexibility.
- Iterate and Improve: Continuous feedback loops and model retraining are vital to keep the AI product improving as new data becomes available.
AI Product Development Phases
- Initial Research and Planning: Understanding market needs and technological constraints.
- Data Collection and Preprocessing: Sourcing, cleaning, and preparing data for training.
- Model Development: Selecting algorithms, training the model, and evaluating performance.
- Deployment: Implementing the AI model in a real-world environment with scalability in mind.
- Continuous Monitoring and Iteration: Regular model updates and performance optimization to ensure sustainability.
Important Considerations for Scalability
Consideration | Description |
---|---|
Data Availability | Ensure that data collection scales with the growing size of the product. |
Model Performance | As data grows, models must maintain or improve accuracy while handling larger datasets. |
Infrastructure Flexibility | Use cloud-based or distributed systems for flexibility and to accommodate scaling needs. |
“The scalability of an AI product isn’t just about handling more data; it’s about ensuring that the model remains efficient and relevant as new challenges arise.”
Understanding the Legal and Ethical Implications of AI
When developing AI-based products or services, it's crucial to comprehend the legal and ethical challenges that come with it. As artificial intelligence evolves, its influence on various sectors increases, leading to a complex landscape of laws and regulations that must be navigated. Entrepreneurs in the AI field must understand these implications to avoid legal pitfalls and build trust with users and stakeholders.
Ethical considerations in AI development often overlap with legal concerns but require distinct attention. AI can introduce significant social, economic, and cultural consequences, making it essential for entrepreneurs to not only comply with existing regulations but also take proactive steps to ensure their technology promotes fairness, transparency, and accountability.
Key Legal Considerations
- Data Privacy Laws: AI systems often rely on vast amounts of personal data, which brings the need for strict adherence to data protection regulations such as GDPR in Europe or CCPA in California.
- Intellectual Property: Ensuring that AI models, datasets, and algorithms do not infringe upon the intellectual property rights of others is critical for avoiding lawsuits and intellectual property theft.
- Liability and Accountability: In case of harm or damage caused by AI systems, understanding who is legally responsible is an ongoing legal challenge that needs to be addressed.
Ethical Challenges
- Bias and Discrimination: AI systems can perpetuate biases present in training data, leading to unfair outcomes. Entrepreneurs must take steps to identify and mitigate bias in their models.
- Transparency and Explainability: AI decisions can be opaque. Ensuring that AI systems provide understandable reasons for their actions is critical for building trust and accountability.
- Autonomy and Control: The ethical dilemma of how much autonomy should be given to AI systems, especially in sensitive fields like healthcare, law enforcement, and finance, is a central concern.
"In the rapidly evolving world of AI, legal and ethical considerations are not just regulatory hurdles but integral parts of building sustainable and responsible businesses."
Table of Relevant Regulations
Regulation | Region | Key Focus |
---|---|---|
GDPR | EU | Data protection, user privacy |
CCPA | California, USA | Consumer rights, data transparency |
AI Act | EU | AI safety, ethical use |
How to Secure Funding for an AI Startup
Securing capital is one of the key steps for any AI startup. Whether you're developing a new machine learning tool or working on a cutting-edge AI-driven service, funding is crucial for scaling operations, hiring talent, and advancing your product. There are multiple funding avenues available to entrepreneurs looking to get their AI startup off the ground. Each comes with its own advantages, but understanding which source best fits your needs is vital.
For an AI startup, the funding process may include a combination of early-stage investments, grants, and partnerships. As the AI field is highly innovative and requires substantial research and development, demonstrating clear value and scalability to potential investors is crucial. Below are the primary ways to secure the necessary funds for launching and growing your AI company.
1. Venture Capital (VC) Funding
Venture capital is one of the most common sources of funding for high-growth startups. VC firms are typically interested in companies that show a high potential for scalability and profitability. To secure VC investment, focus on presenting:
- A clear problem your AI solution addresses.
- A compelling market opportunity with data-backed projections.
- A talented team with expertise in AI and business development.
- A clear go-to-market strategy and timeline for growth.
"Investors want to see your AI startup not only has the technology but also a strategy to bring it to the market and make it profitable." - AI Industry Expert
2. Government Grants and Research Funding
Many governments offer grants to support AI research and development, particularly in areas that align with public interests, such as healthcare, education, or climate change. Here’s what you need to know:
- Explore both local and international programs dedicated to AI development.
- Ensure your project meets the specific eligibility criteria and aligns with the objectives of the grant.
- Prepare a detailed research proposal outlining how your AI technology contributes to the industry or society.
Funding Source | Eligibility | Application Process |
---|---|---|
EU Horizon 2020 | AI-driven projects with societal impact | Submit a detailed project proposal through the EU portal |
SBIR (US) | AI research aligned with federal priorities | Apply via a formal solicitation process |
3. Strategic Partnerships and Corporate Funding
Partnering with established companies in your industry can offer access to both capital and resources. Large corporations, especially those with AI departments, may be willing to fund innovative AI startups in exchange for a partnership or early access to your technology. Keep in mind:
- Ensure there’s a mutual benefit for both parties.
- Maintain control over key intellectual property (IP).
- Negotiate terms clearly to avoid conflicts down the road.
Choosing the Right AI Tools and Frameworks for Your Business
Selecting the right AI tools and frameworks is crucial for the success of your business. With the rapidly evolving landscape of artificial intelligence, it’s important to make informed decisions to avoid wasting resources on inefficient technologies. The right tools can enhance productivity, streamline processes, and give your business a competitive edge.
When choosing AI tools, consider the specific needs of your business. Do you need a tool for natural language processing (NLP), computer vision, or predictive analytics? Each framework offers unique capabilities, so understanding the goals of your business will guide your decision-making process.
Key Factors to Consider
- Scalability: Make sure the tool can grow with your business and handle increasing data volumes.
- Ease of Integration: Look for frameworks that can easily integrate with your existing systems and databases.
- Support and Documentation: Ensure the tools you choose come with strong community support and detailed documentation.
- Cost: Evaluate the pricing structure to avoid overspending on tools that don’t deliver enough value.
AI tools that don't scale effectively or integrate smoothly into your business can become costly and time-consuming. Make sure to choose wisely to avoid these pitfalls.
Popular AI Frameworks and Tools
Tool/Framework | Use Case | Best For |
---|---|---|
TensorFlow | Deep learning and neural networks | Scalable AI applications |
PyTorch | Deep learning and research | Researchers and prototyping |
scikit-learn | Machine learning algorithms | Data science and analytics |
OpenCV | Computer vision tasks | Image and video analysis |
Next Steps for Implementation
- Evaluate the needs: Clearly define the problems you want to solve with AI.
- Test tools: Run pilots with different frameworks to see which fits your requirements.
- Hire experts: Ensure your team has the technical skills to implement the chosen frameworks effectively.
Building a High-Performance AI Team: Key Roles and Skills
Assembling a team capable of executing AI-driven projects requires not only a combination of technical expertise but also strategic alignment with business goals. The ideal AI team blends various skills, ensuring that all aspects of AI research, development, and deployment are covered. A balanced team will typically consist of experts in machine learning, software engineering, data science, and domain-specific knowledge. The following outlines key roles and skills needed for a high-performance AI team.
To build a team that can handle the complexities of AI, it is essential to focus on both technical and collaborative competencies. Each team member should bring a unique set of capabilities to the table, whether it be deep learning algorithms, system architecture, or data analytics. Below are the crucial roles and skill sets needed to create a successful AI development environment.
Key Roles and Required Skills
- Machine Learning Engineer: Focuses on developing and implementing machine learning models. Must be proficient in deep learning, reinforcement learning, and supervised/unsupervised learning techniques.
- Data Scientist: Handles data exploration, feature engineering, and data analysis. Should be skilled in statistics, data visualization, and using libraries like Pandas and NumPy.
- AI Researcher: Conducts cutting-edge research to develop novel algorithms and improve existing AI techniques. A strong foundation in mathematics and theoretical AI is essential.
- Software Engineer: Ensures the scalability and robustness of AI systems. Expertise in programming languages such as Python, C++, or Java is required.
- Product Manager: Bridges the gap between business needs and technical execution. Should have a deep understanding of both AI capabilities and the business goals the technology is serving.
- UX/UI Designer: Creates user-friendly interfaces for AI products. Should have a background in design principles and a solid understanding of user experience in AI-driven solutions.
Essential Skills for High-Performance Teams
- Collaborative Communication: The ability to effectively communicate complex technical concepts to non-technical stakeholders is critical for successful AI projects.
- Adaptability: The AI field is rapidly evolving, so team members must be willing to learn new techniques and adapt to changing technologies.
- Problem-Solving: The AI industry is inherently challenging, and finding innovative solutions to problems is a necessary skill across all roles.
A diverse team with a blend of technical and domain-specific knowledge is the foundation of a successful AI business venture.
Team Structure and Collaboration
Role | Primary Responsibilities | Key Skills |
---|---|---|
Machine Learning Engineer | Develop models, train algorithms, optimize performance | Deep learning, Python, TensorFlow |
Data Scientist | Analyze data, generate insights, perform statistical analysis | Statistics, Pandas, NumPy |
AI Researcher | Conduct research on AI algorithms, enhance model efficiency | Mathematics, Research methods, AI theory |
Software Engineer | Develop and deploy AI systems, ensure scalability | Programming languages, Cloud platforms |
Product Manager | Align business goals with technical solutions, manage project timelines | Leadership, Strategic thinking, AI knowledge |
UX/UI Designer | Design interfaces, focus on user experience of AI products | Design software, User-centric design |
Testing and Iterating Your AI Solution in the Real World
Once your AI product is developed, it's time to test it in a real-world environment. This stage involves more than just running the program on a test set–it requires rigorous testing against dynamic and unpredictable inputs, as well as user interactions. Ensuring that your AI solution performs well under varying conditions is crucial to its success and scalability. Proper testing will also help identify and resolve potential issues early on, saving you time and resources in the long run.
Iteration is equally important. Based on the results from testing, refining the product becomes an ongoing process. Collecting feedback from real users and analyzing their experiences will provide insights into areas where the AI can be improved. Whether it's enhancing the model's accuracy or refining its user interface, continuous iteration is key to staying competitive and meeting evolving customer needs.
Key Steps for Effective Testing and Iteration
- Define Clear Testing Goals: Understand what you want to measure, such as accuracy, speed, or user satisfaction.
- Simulate Real-World Scenarios: Test with real data and in environments that closely resemble how the product will be used.
- Collect User Feedback: Involve real users early on to identify usability issues and unexpected behavior.
- Analyze Data Continuously: Use metrics and user input to guide your next steps and refine your AI model.
Testing and iterating in real-world conditions is not just a phase but a continuous cycle. Every product release should bring meaningful improvements based on collected data and insights.
Common Testing Methods for AI Products
- Beta Testing: Invite a select group of users to test the AI product in its near-final form.
- A/B Testing: Compare different versions of your AI system to see which performs better with real users.
- Performance Testing: Evaluate how well the AI system handles various workloads and data volumes in production environments.
Example Iteration Table
Version | Changes Made | Results |
---|---|---|
1.0 | Initial release with basic functionality. | Successful but lacks accuracy in edge cases. |
1.1 | Improved model accuracy and added new features. | Better performance but still struggles with real-time data. |
1.2 | Enhanced data handling and UI/UX adjustments. | Positive user feedback, better reliability. |