Will Financial Analysts Be Automated
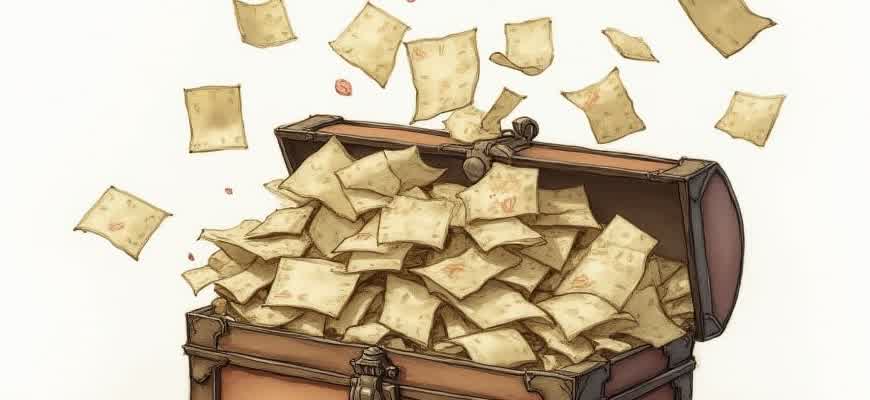
The financial sector has always been at the forefront of technological advancements, with analysts continually seeking ways to improve efficiency and accuracy. As artificial intelligence (AI) and machine learning continue to evolve, many are questioning whether human financial analysts will be replaced by automated systems in the near future.
Financial analysts perform tasks such as evaluating market trends, forecasting economic performance, and advising businesses on investment strategies. While these tasks require both technical skills and a deep understanding of market behavior, recent developments in AI suggest that automation could handle many of these responsibilities. Some key factors influencing this shift include:
- The growing sophistication of AI algorithms in data processing.
- Increased availability of big data and the ability to analyze it rapidly.
- The rise of automated financial tools and platforms used by businesses and investors.
"While automation can enhance data analysis, the human touch remains crucial for interpreting complex financial scenarios and offering strategic guidance."
Despite the advancements, it is important to consider which aspects of financial analysis are most susceptible to automation. Below is a breakdown of tasks that could be automated versus those that require human intervention:
Task | Potential for Automation |
---|---|
Market trend analysis | High |
Investment strategy development | Moderate |
Client relationship management | Low |
How AI is Impacting Financial Data Analysis Today
Artificial intelligence is revolutionizing the way financial analysts process and interpret data. With its ability to quickly analyze vast amounts of financial data, AI is becoming a critical tool in decision-making processes. The traditional methods of financial analysis, which rely heavily on manual data input and subjective interpretation, are being replaced by machine learning models that can identify patterns, predict trends, and optimize investment strategies more efficiently.
AI-powered tools are not only improving the speed of data processing but also enhancing the accuracy of predictions. These systems are capable of processing unstructured data such as news articles, social media feeds, and financial reports, making it easier to assess market sentiment and forecast price movements. The increasing use of AI in financial data analysis is transforming the entire industry, providing analysts with more reliable insights and reducing the margin for human error.
Key Impacts of AI on Financial Data Analysis
- Automation of Repetitive Tasks: AI tools automate the most tedious aspects of data collection, cleaning, and analysis, freeing analysts to focus on strategic decision-making.
- Predictive Analytics: Machine learning algorithms can predict future market trends by analyzing historical data and identifying correlations that humans might miss.
- Sentiment Analysis: AI can analyze massive amounts of textual data, including news articles and social media, to gauge market sentiment and adjust forecasts accordingly.
How AI Enhances Data-Driven Decisions
AI does not just assist analysts; it actively augments their decision-making by providing data-driven insights that were previously impossible to obtain manually.
- Risk Management: AI models help financial analysts assess risks more accurately by evaluating a wider range of factors and predicting potential adverse events.
- Improved Forecasting: Machine learning algorithms continuously refine their predictions based on new data, allowing for more accurate long-term financial forecasts.
- Operational Efficiency: AI optimizes workflows by automating data collection and analysis, resulting in faster decision-making and reduced operational costs.
AI-Driven Tools in Use Today
Tool | Application | Impact |
---|---|---|
Robo-Advisors | Automated investment advice based on client data. | Improved accessibility and personalized investment strategies. |
Natural Language Processing (NLP) | Analyzing unstructured text data such as earnings reports and news articles. | Enhanced ability to extract actionable insights from diverse data sources. |
Predictive Analytics Platforms | Analyzing historical financial data to forecast future market movements. | Increased accuracy in predicting stock prices and economic trends. |
Which Financial Analyst Tasks Are Most Likely to Be Automated?
With the rapid development of artificial intelligence (AI) and machine learning technologies, financial analysts face increasing pressure to adapt to automated processes. Many routine tasks that were once time-consuming and repetitive are now being taken over by advanced algorithms. These tasks are often related to data processing, pattern recognition, and predictive modeling, areas where AI can significantly enhance efficiency.
While some aspects of financial analysis require deep human judgment, numerous functions lend themselves well to automation. Tasks such as data collection, report generation, and forecasting can be performed by AI with greater accuracy and speed than a human analyst. Below are some specific tasks most likely to be automated:
1. Data Collection and Processing
Financial analysts spend a large portion of their time gathering and organizing financial data from multiple sources. AI tools can easily automate this task by using web scraping, data extraction algorithms, and APIs to pull information from financial statements, market feeds, and news outlets.
- Gathering stock prices, interest rates, and economic indicators
- Scraping financial reports and earnings calls
- Consolidating data from various financial systems
2. Report Generation
Creating financial reports is another time-consuming task that is increasingly being automated. AI can generate reports by processing raw data and summarizing findings in a format that is ready for review. These reports can range from daily financial summaries to more complex quarterly or annual reports.
"AI can improve the speed of report generation while ensuring data accuracy and consistency."
- Daily financial summaries
- Investment performance reports
- Risk analysis and mitigation reports
3. Forecasting and Predictive Analytics
AI's ability to process vast amounts of historical data makes it ideal for generating forecasts. Machine learning models can analyze trends, seasonality, and market sentiment to make predictions about stock movements, interest rate changes, and economic cycles.
Task | Automation Potential |
---|---|
Stock price predictions | High |
Credit risk assessment | High |
Economic forecasting | Medium |
Automation Tools: What’s Available for Financial Analysts Now?
As the demand for increased efficiency in financial analysis grows, a variety of automation tools have emerged to assist financial analysts. These tools are designed to reduce manual labor, increase accuracy, and provide real-time insights, allowing professionals to focus more on strategic decision-making. The use of artificial intelligence, machine learning, and advanced data analytics is quickly becoming an integral part of financial analysis workflows.
Currently, financial analysts have access to numerous software solutions, each catering to different aspects of financial analysis. These tools are primarily aimed at automating data collection, analysis, and reporting, allowing analysts to make informed decisions faster and with greater precision.
Common Automation Tools for Financial Analysts
- RPA (Robotic Process Automation): Tools like UiPath and Automation Anywhere are popular for automating repetitive tasks such as data entry, report generation, and invoice processing.
- Financial Modelling Software: Solutions like Quantrix and Adaptive Insights automate complex financial modeling, allowing analysts to visualize data and perform simulations with ease.
- AI-Driven Analytics: Platforms like Alteryx and Tableau use machine learning algorithms to analyze large datasets, generating insights that would take analysts much longer to uncover manually.
- Forecasting Tools: Tools such as IBM Watson and Prophet are designed to predict market trends based on historical data, enabling analysts to adjust forecasts quickly and efficiently.
"Automation tools in financial analysis enable analysts to focus on higher-value tasks such as strategy development and risk management, instead of spending time on data collection and routine calculations."
Comparing Key Automation Platforms
Tool | Key Feature | Best For |
---|---|---|
UiPath | Robotic Process Automation (RPA) | Automating repetitive tasks like data entry and report generation |
Alteryx | AI and machine learning analytics | Advanced data analytics and insight generation |
Adaptive Insights | Financial planning and forecasting | Budgeting, planning, and financial reporting |
IBM Watson | Predictive analytics and forecasting | Forecasting market trends and financial predictions |
Impact of Automation on the Finance Job Market
The advent of automation in finance brings significant transformations to the job market. As technology continues to evolve, many traditional finance roles are expected to undergo substantial changes. Tasks that once required human intervention, such as data analysis, forecasting, and report generation, are increasingly being performed by advanced algorithms and machine learning models. This shift poses both opportunities and challenges for the workforce in finance.
Automation will likely redefine the skill sets needed in the industry, potentially displacing some jobs while creating new opportunities. The demand for certain roles may diminish as automated systems handle repetitive and rule-based tasks. However, automation will also spur the growth of roles that require higher-level thinking, innovation, and human judgment, especially in areas that machines cannot replicate easily, such as strategic decision-making and relationship management.
Effects on Employment in the Finance Sector
Key Changes in Job Roles:
- Reduction of routine administrative roles, such as bookkeeping and transaction processing, due to automated software.
- Growth of roles focused on managing and interpreting machine-generated insights, including data scientists and AI specialists.
- Increased importance of professionals capable of overseeing automated systems and ensuring their alignment with business strategies.
New Skill Requirements:
- Advanced technical skills, such as proficiency in machine learning, artificial intelligence, and data analytics.
- Strong business acumen to translate automated insights into actionable strategies.
- Improved soft skills for relationship management, as personal interactions will still be crucial in advisory roles.
Automation in finance can bring efficiency and cost savings, but the human element remains essential for interpreting complex data and making nuanced decisions.
Impact on Various Finance Job Categories
Job Category | Impact of Automation |
---|---|
Financial Analysts | Routine tasks like data collection and preliminary analysis will be automated, reducing manual workloads. |
Investment Bankers | Automation will streamline data processing and market analysis, but personal client relationships will still require human involvement. |
Accountants | Automation can handle basic bookkeeping and compliance tasks, but strategic financial planning will need human oversight. |
How Financial Analysts Can Adapt to an Automated Future
The rapid rise of automation in finance presents a challenge for financial analysts who traditionally rely on human judgment and experience. With algorithms capable of analyzing large datasets faster than humans, analysts must evolve their skill sets to remain competitive. Automation can handle routine tasks such as data collection and preliminary analysis, but analysts can shift their focus to areas where human expertise is still crucial, such as interpretation of complex financial patterns and decision-making processes.
To thrive in this evolving environment, financial analysts should prioritize developing skills that complement automation. The ability to leverage new technologies and adapt to AI-driven tools will be essential. Analysts who can integrate advanced analytics into their workflows while maintaining critical thinking will continue to provide significant value to organizations. Below are key strategies for financial analysts to adapt to an automated future.
Key Strategies for Adapting
- Focus on Critical Thinking and Problem Solving: While automation can handle repetitive tasks, human judgment is still needed for interpreting data in complex scenarios. Analysts should hone their ability to provide strategic insights based on data.
- Learn New Technologies: Familiarity with tools like machine learning models, predictive analytics, and AI-based financial platforms will help analysts stay relevant. Continually upgrading technical skills will be crucial for adapting to automation.
- Improve Soft Skills: As automation reduces the need for routine tasks, communication, leadership, and negotiation will become more important. Analysts should focus on developing interpersonal and management skills to guide teams and clients through complex decisions.
New Roles for Financial Analysts
- Data Curators: Financial analysts can step into roles focused on ensuring data integrity, managing the input for AI systems, and refining the outputs to ensure accuracy and relevancy.
- AI Trainers: Analysts can work closely with machine learning models to help them learn and evolve, providing feedback and human insights to improve the accuracy of predictions.
- Decision Support Specialists: Analysts can shift to providing higher-level decision-making support by interpreting AI outputs and translating them into actionable strategies for businesses.
Essential Skills for the Future
Skill | Importance | How to Develop |
---|---|---|
Advanced Data Analysis | High | Enroll in courses on data science and analytics, practice with real-world data sets. |
AI & Machine Learning Understanding | Medium | Take online courses, participate in industry webinars, and use AI tools in financial models. |
Strategic Thinking | High | Work closely with senior analysts and business leaders to understand the broader context of financial decisions. |
"The key to staying relevant in an automated world is not to fight against technology, but to embrace it and use it to your advantage."
The Role of Human Judgment in a Data-Driven Financial World
In the age of advanced analytics and artificial intelligence, the role of human decision-making remains indispensable in the financial sector. While algorithms can process vast amounts of data and identify patterns with impressive speed, human judgment is crucial in interpreting complex situations where data alone cannot provide a full picture. Financial analysts, with their experience and intuition, are often required to evaluate scenarios that involve uncertainty, emotions, or regulatory nuances, which machines cannot yet fully grasp.
The increasing reliance on data-driven tools raises an important question: can technology replace human insight in making critical financial decisions? While automation can certainly improve efficiency and reduce human error, it is human judgment that provides the nuanced perspective necessary for managing risks and navigating unforeseen market conditions.
Why Human Insight Matters
Human analysts bring several key elements to the table that technology lacks:
- Contextual Understanding: Analysts can interpret the broader economic environment and company culture, which algorithms may overlook.
- Ethical Considerations: Decisions in finance often require a human touch to ensure they align with ethical standards, something AI cannot always prioritize.
- Adaptability: Humans can quickly adjust to changing circumstances, while automated systems may require retraining or significant reprogramming.
Limitations of Data-Driven Models
Despite the benefits, data-driven financial models have inherent limitations:
- Bias in Data: If the underlying data contains biases, the model’s predictions can be skewed, leading to flawed decisions.
- Uncertainty in Markets: Financial markets are often influenced by unpredictable human behavior and geopolitical events, factors difficult for machines to account for.
- Lack of Emotional Intelligence: AI cannot replicate the emotional intelligence that humans apply when gauging market sentiment or managing client relationships.
Key Takeaways
Aspect | Human Judgment | Automated Systems |
---|---|---|
Data Processing | Analyzes data with context and intuition | Processes large datasets quickly but lacks context |
Adaptability | Quickly adapts to unforeseen events | Requires retraining or manual intervention |
Ethical Decisions | Considers ethical implications and values | Lacks an ethical framework |
"While technology accelerates efficiency, human judgment ensures that decisions are made with the necessary nuance, creativity, and foresight."
How AI Can Improve Accuracy and Speed in Financial Reporting
Financial reporting is an essential function in the financial industry, but it is often time-consuming and prone to human error. Artificial Intelligence (AI) can help eliminate many of these challenges by automating data processing and analysis. This allows financial reports to be generated more quickly, with greater precision. AI-driven systems are capable of analyzing vast amounts of data in real time, enabling more accurate forecasting and reporting. The speed at which these systems work means that companies can receive insights faster and make informed decisions without delay.
One of the key benefits of AI in financial reporting is its ability to reduce errors that are often caused by manual data entry and calculations. By leveraging machine learning algorithms and advanced data analytics, AI systems can identify patterns, spot discrepancies, and correct mistakes that might otherwise go unnoticed. The result is more reliable financial statements and reduced risk for businesses.
Key Benefits of AI in Financial Reporting
- Faster Data Processing: AI can process large volumes of financial data in seconds, allowing reports to be produced almost instantaneously.
- Improved Accuracy: By automating the data input process, AI minimizes the potential for human error, ensuring more accurate financial reports.
- Real-Time Insights: AI can provide up-to-date financial insights, enabling businesses to make quicker, more informed decisions.
- Enhanced Decision Making: With more reliable data, decision-makers can rely on AI-generated reports to guide their financial strategies.
Examples of AI Tools in Financial Reporting
- AI-powered data aggregation tools that collect and compile financial data from various sources.
- Machine learning models that analyze past financial trends and predict future outcomes.
- Automated reconciliation software that compares financial data across different platforms and identifies inconsistencies.
Impact on Financial Reports
"AI-driven reporting systems help eliminate the subjectivity and bias that can arise from human interpretation of financial data, leading to more objective and reliable reports."
Benefit | Description |
---|---|
Efficiency | Reduces the time needed to produce financial reports, freeing up resources for other tasks. |
Consistency | AI ensures that reports are created using the same methodology, minimizing variability. |
Risk Reduction | By detecting discrepancies early, AI helps mitigate risks associated with inaccurate financial reporting. |