Ai Business Challenge
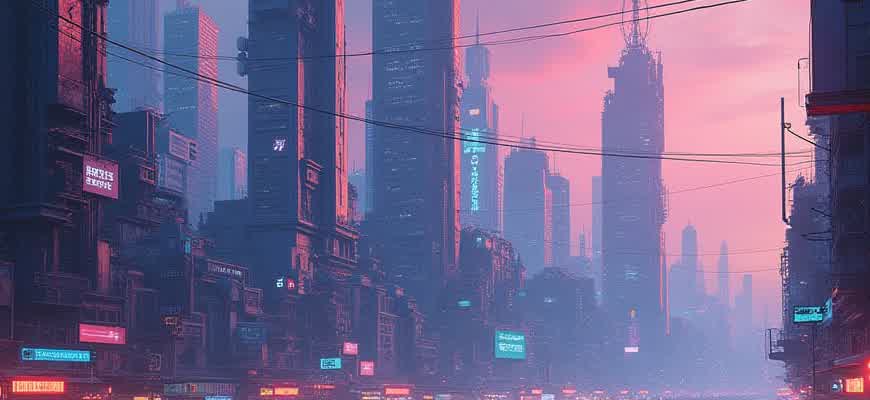
The integration of artificial intelligence into business operations offers numerous opportunities but also presents unique challenges. One of the primary hurdles is the complexity of adopting AI technologies and aligning them with existing business strategies. Companies face difficulties in scaling AI solutions and ensuring that they provide measurable value across various departments.
To effectively implement AI, organizations must consider several critical factors:
- Data quality and availability
- Alignment of AI initiatives with business goals
- Ensuring transparency and accountability in decision-making
- Investing in upskilling employees for AI-related tasks
In response to these challenges, businesses are adopting specific approaches to navigate the AI landscape:
- Establishing clear objectives for AI initiatives
- Implementing cross-functional teams for better collaboration
- Prioritizing data governance and privacy
"AI can revolutionize industries, but only if businesses are willing to adapt and face the inherent challenges in its implementation."
Table 1 below highlights the core areas where companies typically encounter difficulties when integrating AI solutions:
Challenge | Description | Impact |
---|---|---|
Data Management | Accessing and organizing large volumes of data for AI processing | Slow AI adoption, inconsistent results |
Talent Acquisition | Finding skilled professionals with AI expertise | Higher recruitment costs, delayed project timelines |
Integration Complexity | Integrating AI systems into existing infrastructure | Increased operational disruption, longer implementation time |
Harnessing AI Innovation for Business Growth
As businesses seek to stay competitive in an increasingly digital world, leveraging AI technologies has become a crucial strategy. AI-driven solutions offer unprecedented capabilities, from automation of repetitive tasks to predictive analytics that drive smarter decision-making. In industries ranging from finance to healthcare, AI is unlocking new revenue streams and improving operational efficiency.
Embracing cutting-edge AI technology is not just about staying ahead of competitors–it's about transforming business models for long-term sustainability. Through the integration of machine learning, natural language processing, and data analytics, organizations can create personalized customer experiences, enhance product development, and streamline supply chain operations.
Key Benefits of AI-Driven Business Transformation
- Automation of Processes: AI helps reduce the need for manual intervention in routine tasks, leading to faster execution and reduced errors.
- Enhanced Data Analysis: AI-powered analytics tools provide businesses with deeper insights from large volumes of data, aiding in more accurate forecasting and decision-making.
- Improved Customer Experience: AI enables personalized customer interactions by analyzing behavior patterns and preferences.
Steps to Implement AI in Business Strategy
- Assess Business Needs: Identify areas where AI can add the most value, such as customer service, marketing, or logistics.
- Invest in the Right Tools: Choose AI solutions that align with the company’s objectives, such as chatbots for customer support or AI-driven analytics for sales optimization.
- Train Employees: Equip teams with the necessary skills to work alongside AI systems effectively.
- Measure and Optimize: Continuously monitor the impact of AI technologies and refine strategies based on performance data.
"AI adoption is no longer a futuristic concept; it’s a key driver of business growth and innovation today."
AI Technologies Transforming Industries
Industry | AI Application | Impact |
---|---|---|
Healthcare | Predictive Diagnostics | Faster, more accurate diagnoses that lead to better patient outcomes. |
Retail | Personalized Recommendations | Improved customer satisfaction and higher conversion rates. |
Finance | Fraud Detection | Increased security and reduced financial losses due to fraud. |
How AI-Driven Competitions Reveal New Market Insights
AI business challenges offer a structured environment where innovators can address real-world problems using cutting-edge technologies. These competitions encourage participants to focus on identifying gaps in current markets and create solutions that could transform industries. By analyzing large datasets, testing new algorithms, and developing prototypes, participants often uncover unmet needs or areas with significant growth potential.
As these challenges require both technical expertise and strategic thinking, participants gain valuable insights into consumer behavior, industry trends, and emerging technologies. In turn, this helps identify new opportunities that may not be immediately obvious in traditional market research.
Key Advantages of AI Business Challenges in Identifying Opportunities
- Data-Driven Insights: Competitions encourage the analysis of massive datasets, revealing trends and customer needs that may otherwise go unnoticed.
- Competitive Edge: By focusing on innovative AI solutions, participants can spot market gaps where existing businesses fail to address specific demands.
- Cross-Industry Applications: The versatility of AI allows teams to explore opportunities across various sectors, from healthcare to finance, helping uncover potential in underserved industries.
“AI business challenges push teams to think outside the box, helping identify areas for disruption where innovation can lead to significant market shifts.”
Examples of Identifying Opportunities
Industry | Identified Opportunity | AI Solution |
---|---|---|
Healthcare | Predictive analytics for patient outcomes | Machine learning models trained on health records |
Retail | Personalized customer experiences | AI-driven recommendation systems |
Finance | Fraud detection and prevention | AI algorithms for real-time transaction monitoring |
Steps to Implement AI-Driven Solutions for Your Business
Integrating AI technology into your business processes can significantly boost efficiency, decision-making, and customer engagement. However, the journey from idea to execution requires careful planning and the right steps. This guide outlines the core actions needed to successfully launch an AI-powered solution within your organization.
To ensure smooth implementation, you need a clear strategy, starting with understanding your business challenges and how AI can address them. Once you've identified the problem areas, the following steps will guide you through the process of deploying an AI-driven solution effectively.
Key Phases of AI Solution Deployment
- Identify the Business Need: Analyze your business operations and pinpoint tasks or processes that could benefit from automation or enhanced decision-making through AI.
- Choose the Right AI Technology: Evaluate different AI technologies (machine learning, natural language processing, etc.) to determine which one aligns with your needs.
- Data Collection and Preparation: Gather high-quality, relevant data. Data cleaning and preprocessing are essential for training AI models effectively.
- Develop and Train the Model: Work with data scientists to build, train, and fine-tune your AI models for specific use cases.
- Integration and Testing: Integrate the AI solution into your existing infrastructure. Perform rigorous testing to ensure compatibility and performance.
- Monitor and Optimize: Once deployed, continuously monitor the AI system's performance and make adjustments to optimize outcomes.
Important: Ensure that your team has the technical expertise to manage and maintain the AI solution. Inadequate support can lead to failures in the long term.
Table of Considerations for AI Solution Selection
Factor | Consideration |
---|---|
Budget | Determine how much you're willing to invest in AI, considering both development and ongoing maintenance costs. |
Scalability | Choose solutions that can scale with your business growth and adapt to new challenges. |
Data Availability | Ensure you have access to the necessary data for AI training and real-time decision-making. |
Integration Capabilities | Verify that the AI solution can integrate seamlessly with your current business systems. |
Key Takeaways
- Identify a clear business challenge where AI can add value.
- Ensure data quality and prepare for rigorous model training.
- Monitor and continuously improve AI solutions to keep them aligned with business needs.
How to Assess the Impact of AI Solutions on Financial Performance
Measuring the effect of AI tools on business performance requires a clear framework that links technology deployment with business outcomes. In many cases, the return on investment (ROI) can be elusive, but it is critical to capture the right metrics to ensure AI solutions deliver value. The evaluation process should involve financial and operational indicators, such as revenue growth, cost reduction, and productivity improvements, while also considering the non-financial benefits like customer satisfaction or brand enhancement.
The success of AI implementation is not only about direct monetary gains. To understand its real impact, businesses must focus on key performance indicators (KPIs) that reflect both short-term and long-term results. By analyzing these metrics, companies can determine whether AI technologies are generating sustainable improvements to their bottom line and identify areas for further optimization.
Key Metrics for Measuring AI's Impact
- Revenue Growth: Track sales increase, new market penetration, or customer acquisition linked to AI-driven efforts.
- Operational Efficiency: Measure reductions in process time, resource usage, or manual labor costs.
- Customer Experience: Use satisfaction surveys, retention rates, and engagement metrics to gauge AI's effect on customer loyalty.
- Cost Reduction: Monitor savings from automation or smarter resource allocation powered by AI.
Quantitative Methods for Measuring AI ROI
- Cost-Benefit Analysis: Compare the total cost of AI deployment (including hardware, software, and training) with the financial returns achieved.
- Time-to-Value: Track how quickly AI tools contribute to meeting business goals or financial targets.
- Impact on Productivity: Calculate efficiency gains by measuring output per employee or task completion time.
"To achieve meaningful results, businesses must align AI applications with their strategic objectives, ensuring that every implementation directly ties into measurable outcomes that contribute to the company’s financial health."
Evaluating Long-Term Effects of AI
In addition to immediate benefits, it's crucial to assess the long-term effects of AI on market positioning and innovation capacity. This can be done by tracking competitive advantage, brand recognition, and the ability to scale operations without proportional increases in costs.
Metric | Short-Term Impact | Long-Term Impact |
---|---|---|
Revenue Growth | Increased sales from AI-driven campaigns | Establishment of sustainable revenue streams through personalized services |
Cost Efficiency | Immediate savings in labor and operational costs | Continuous reductions in overhead as AI optimizes more processes |
Customer Loyalty | Enhanced experience through AI-powered recommendations | Long-term relationships driven by AI-based personalization |
Maximizing ROI through AI Integration: Practical Strategies for Business
Integrating Artificial Intelligence into business processes can drastically enhance productivity and reduce operational costs. The key to leveraging AI for maximizing return on investment (ROI) lies in identifying the right areas within a company where AI can add value. AI is not just a tool for automation; it can transform decision-making, customer engagement, and even internal workflows.
Successful AI integration requires a clear strategy focused on long-term results. Companies must approach AI as an enabler, rather than a one-time investment. By embedding AI throughout key operations, businesses can see incremental improvements that collectively lead to substantial ROI over time.
Effective Strategies for AI Adoption
- Data-Driven Decision Making: Utilize AI's predictive capabilities to analyze customer data and market trends for more informed business decisions.
- Customer Experience Optimization: Use AI-powered chatbots and recommendation systems to improve customer engagement and retention.
- Operational Efficiency: Implement AI to automate routine tasks and improve internal workflows, freeing up resources for more strategic initiatives.
- Cost Reduction: By replacing manual processes with AI-driven automation, businesses can achieve substantial savings on labor and operational costs.
Key Factors for Maximizing ROI with AI
- Continuous Improvement: AI models should be constantly refined based on new data and insights to ensure they remain effective and relevant.
- Employee Training: Equip your workforce with the necessary skills to work alongside AI technologies, fostering collaboration rather than resistance.
- Scalable Solutions: Ensure that AI systems are scalable to accommodate future growth and increasing amounts of data.
- Clear KPIs: Establish clear performance indicators to track the success of AI initiatives and assess their impact on business outcomes.
"The true value of AI comes not just from its ability to perform tasks, but from its potential to transform the way businesses operate and deliver value to their customers."
AI Integration: Areas of Impact
Business Area | AI Application | Expected Benefit |
---|---|---|
Marketing | AI-driven analytics for customer segmentation | Improved targeting and higher conversion rates |
Customer Service | AI-powered chatbots and virtual assistants | Enhanced customer satisfaction and reduced response times |
Finance | AI for fraud detection and risk management | Reduced losses and improved decision-making |
Common Pitfalls in AI Adoption and How to Avoid Them
Implementing AI into a business operation can significantly enhance efficiency, but the process is often riddled with challenges. Many organizations dive into AI without fully understanding its complexities, leading to implementation failures. Recognizing common obstacles early on can help businesses take the necessary precautions to avoid them, ensuring a smoother integration process.
From a lack of clear objectives to inadequate data, several issues arise during AI adoption. Understanding these pitfalls is crucial for any organization that wishes to leverage AI's potential while minimizing risks.
1. Undefined Goals and Misalignment with Business Objectives
One of the most significant challenges businesses face when integrating AI is not having clear and measurable goals. Without a defined purpose, AI solutions can become misaligned with the company’s overall strategy. This lack of direction leads to inefficiencies and missed opportunities.
Ensure your AI strategy aligns with the broader business goals. AI should not be a solution looking for a problem; it should directly address specific, measurable business outcomes.
- Start by identifying clear business objectives that AI can help achieve.
- Set measurable KPIs to track progress and success.
- Involve stakeholders across different departments to ensure alignment and understanding.
2. Insufficient or Poor-Quality Data
AI systems rely heavily on data, and its quality is paramount to success. Insufficient data or data that is biased or incorrect can lead to inaccurate AI models, rendering the technology ineffective. Collecting and preparing quality data should be a priority before any AI project is launched.
Invest time in cleaning and validating your data. Garbage-in, garbage-out applies directly to AI systems.
- Ensure data is representative, accurate, and comprehensive.
- Establish data governance practices to ensure ongoing data quality.
- Integrate data from various sources to increase the breadth of the AI model.
3. Overestimating AI Capabilities and Underestimating Costs
There is often a gap between expectations and reality when it comes to AI's capabilities. Many organizations expect AI to deliver instant results without understanding the time and resources required to build, test, and deploy an AI solution. This mismatch can lead to disappointment and financial losses.
Pitfall | Solution |
---|---|
Overestimating AI’s potential | Have realistic expectations and pilot AI projects before full-scale deployment. |
Underestimating implementation costs | Budget for both the technical and human resources needed for a successful implementation. |
Building a Scalable AI Model for Long-Term Success
Creating a robust and scalable AI solution requires a deep understanding of both technical foundations and business needs. It is crucial to design the model in a way that allows for continuous learning and adaptation, ensuring it remains relevant and efficient as the business evolves. The foundation of a successful AI system lies not just in the algorithms used but also in the architecture and infrastructure that supports it, which must be flexible to scale and adjust over time.
To achieve long-term success with an AI model, it is important to consider key factors like data quality, model monitoring, and the ability to iterate. Building with scalability in mind ensures that the model can grow alongside the company and handle increasing complexity and volume of data. The process involves careful planning, strategic investment, and continuous refinement based on real-time insights.
Key Steps for Creating a Scalable AI Model
- Data Preparation and Integration: Ensuring data consistency and accessibility from multiple sources is the first step. Proper data pipelines and integration frameworks are critical.
- Model Design and Flexibility: The model should be modular and easy to update, ensuring that improvements or new features can be added without major rework.
- Infrastructure Scaling: Utilize cloud technologies or hybrid solutions to provide scalable computing resources as needed.
- Performance Monitoring and Feedback: Regular monitoring ensures the model’s outputs are aligned with business goals. A feedback loop enables continuous improvements.
Important Considerations for Long-Term Sustainability
Developing a scalable AI solution is an ongoing process. It involves not just the initial implementation, but also constant evolution and adaptation to new challenges.
- Automation: Automating repetitive tasks improves efficiency and allows resources to focus on more strategic aspects.
- Model Retraining: The AI model should evolve with the business, retraining with new data to adapt to market changes.
- Resource Management: Ensuring that both human and computational resources are aligned with model growth is critical for sustainability.
Resource Allocation and Performance Metrics
Resource | Key Metrics | Action Plan |
---|---|---|
Data | Quality, Volume, Relevance | Invest in data collection and cleaning techniques. |
Computational Resources | Processing Power, Efficiency | Utilize cloud or hybrid infrastructure for scalable resources. |
Model Performance | Accuracy, Speed, Adaptability | Regular monitoring and retraining with new data. |
How AI-Driven Business Competitions Enhance Operational Efficiency
AI-based business challenges have proven to be an effective tool for improving operational efficiency in organizations. By leveraging machine learning models, businesses can streamline workflows, reduce manual labor, and optimize decision-making. These competitions provide a platform for innovative solutions to real-world problems, demonstrating how AI can address specific operational bottlenecks, such as resource allocation, process automation, and data analysis.
In the context of AI business challenges, companies can tap into the power of AI to identify hidden inefficiencies and implement solutions that can drive substantial improvements in performance. Participants often explore areas such as predictive analytics, task automation, and intelligent optimization strategies, which are all essential in enhancing overall productivity. This not only reduces the operational costs but also accelerates the decision-making processes in fast-paced business environments.
Key Benefits of AI Business Challenges in Optimizing Operations
- Improved Decision-Making: AI helps businesses analyze large datasets quickly, providing actionable insights that enable faster, more accurate decisions.
- Process Automation: By automating repetitive tasks, AI solutions reduce human error and free up resources for more strategic activities.
- Predictive Analytics: AI models predict future trends, enabling companies to proactively address issues before they become problems.
Some Examples of Operational Efficiency Gains:
- Resource allocation optimization
- Supply chain management improvements
- Customer service automation
"AI challenges create a unique opportunity to rethink operational processes and explore innovative solutions that can significantly improve productivity and cost-efficiency."
Area | Impact |
---|---|
Automation | Reduction in labor costs, faster task completion |
Data Analysis | Faster insights, better decision-making |
Resource Management | Optimized use of available resources, lower overhead |