How Do Ai Startups Make Money
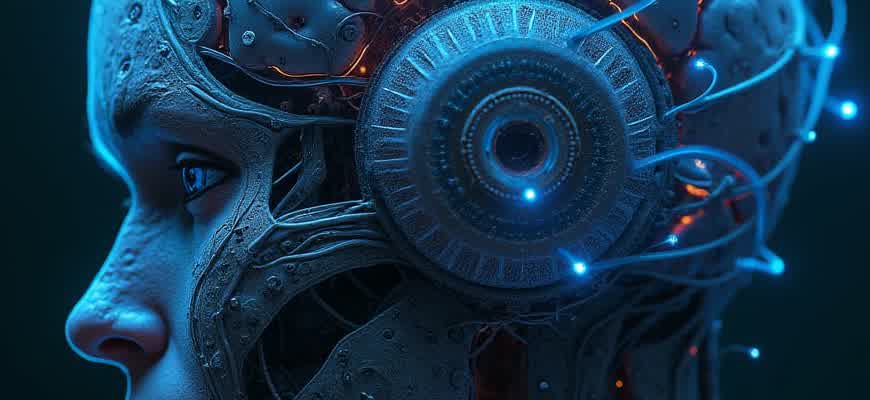
Artificial Intelligence (AI) startups have been growing rapidly in recent years, with many turning innovative solutions into profitable business models. These companies typically generate income through a variety of channels, leveraging their unique technology and data-driven products. Below are the primary methods AI startups use to monetize their offerings:
- Subscription-based Services: Many AI startups offer software as a service (SaaS) products where clients pay for access to AI tools and platforms on a recurring basis.
- Licensing Technology: Some startups license their AI algorithms or platforms to larger companies, generating revenue by allowing others to integrate their technology into existing systems.
- Data Monetization: AI startups can sell valuable data insights or allow third parties to access their data for analysis and decision-making.
AI companies often combine these revenue models to optimize earnings and reduce risk. Additionally, funding from venture capitalists and angel investors plays a crucial role in scaling operations and accelerating growth. Below is a table summarizing these primary revenue strategies:
Revenue Model | Description | Examples |
---|---|---|
Subscription-based | Customers pay a regular fee for continued access to AI-powered tools and services. | Cloud AI platforms like IBM Watson |
Licensing | Companies pay to use or integrate proprietary AI technology into their systems. | AI algorithms used by large corporations |
Data Monetization | Revenue generated by selling or renting valuable data insights and analytics. | Data brokers, market research firms |
AI startups that successfully combine these methods often see rapid growth, driven by both innovation and strategic partnerships.
How AI Startups Generate Revenue
AI startups typically create revenue through a combination of offering specialized solutions, leveraging data, and scaling their services for different industries. Their monetization models vary, but the common thread is the ability to provide AI-driven innovations that solve complex problems more efficiently than traditional methods. These companies often tap into vertical markets such as healthcare, finance, and e-commerce, where AI solutions are in high demand.
Additionally, AI startups often adopt several business models, including subscription-based services, licensing, and performance-based fees. Understanding the value their technology brings to the table allows them to develop targeted revenue streams that appeal to both small businesses and large enterprises. Below are some of the key ways AI startups generate revenue:
- Software as a Service (SaaS): Offering cloud-based AI tools on a subscription model, where users pay regularly for access to the service.
- Licensing Technology: Licensing proprietary AI algorithms or models to other businesses for integration into their products.
- Custom Solutions: Developing tailored AI systems for businesses, often on a project basis, and charging for the design, implementation, and ongoing support.
- Data Monetization: Selling valuable data or insights generated through AI algorithms to third parties.
Blockbuster Example: Many AI startups initially rely on venture capital to fund product development, with plans to scale their revenue as soon as their AI solution proves scalable and effective. This model works well for those targeting complex verticals where competition is low but demand is growing rapidly.
Monetization Models in Detail
Model | Description | Pros | Cons |
---|---|---|---|
Subscription-Based | Regular payments for access to software or tools hosted on the cloud. | Stable revenue stream, high scalability | Dependency on customer retention, churn risk |
Licensing | Granting access to AI technology for integration into third-party products. | High-profit margins, low ongoing costs | Requires a proven track record and strong intellectual property protections |
Custom Development | Creating bespoke AI solutions for specific client needs. | High fees, tailored solutions increase customer loyalty | Time-consuming, may limit scalability |
"AI startups that find success often combine different monetization strategies to diversify their income streams, ensuring a buffer against market volatility."
Identifying Lucrative AI Applications in Specialized Markets
In the rapidly evolving AI landscape, the key to building a profitable AI startup lies in identifying specific, high-value use cases within niche markets. By targeting underserved industries, companies can leverage advanced AI technologies to solve unique problems, thereby gaining a competitive edge. Rather than competing in crowded, generalized sectors, focusing on a well-defined market can lead to quicker adoption and higher customer retention.
The process of identifying valuable AI opportunities starts with understanding the unique needs of these smaller markets. Startups must conduct thorough research to pinpoint challenges that can be solved efficiently with AI, ensuring that the solution not only addresses a pain point but also has a clear path to monetization. Below are some strategies for identifying profitable AI applications in niche markets.
Key Strategies for Identifying Profitable AI Use Cases
- Market Research: Deep analysis of industry-specific challenges can uncover gaps that AI is uniquely positioned to fill. This involves talking to industry experts, reviewing case studies, and analyzing trends.
- Targeting Operational Inefficiencies: AI can automate time-consuming, repetitive tasks in niche sectors, significantly improving operational efficiency.
- Custom AI Solutions: Developing tailored AI tools that directly meet the specific needs of a niche market can provide significant value. For example, AI-driven supply chain optimization tools for small manufacturers.
Potential Niche AI Applications
- Healthcare: AI applications in niche medical fields, such as personalized medicine, are growing rapidly. AI-driven diagnostics tools focused on rare diseases or medical conditions are examples of high-value areas.
- Finance: AI solutions tailored for small financial institutions, such as credit scoring models for underserved demographics, can provide a competitive advantage in the fintech space.
- Manufacturing: Predictive maintenance and AI-based quality control systems for niche manufacturing processes can significantly reduce costs and increase efficiency.
When targeting niche markets, startups must ensure their AI solutions offer measurable outcomes, such as cost reductions or process improvements, to attract investment and ensure long-term profitability.
Example: AI in Precision Agriculture
Sector | AI Application | Value Proposition |
---|---|---|
Agriculture | Precision farming tools, such as AI-based crop monitoring and automated irrigation systems. | Increases crop yields, reduces water waste, and enhances sustainability. |
Healthcare | AI-powered telemedicine platforms offering specialized consultations for rural areas. | Improves access to healthcare in underserved regions, reducing patient wait times. |
Monetizing AI Solutions Through B2B SaaS Platforms
AI-driven startups are increasingly turning to Software as a Service (SaaS) models to monetize their products by offering scalable, subscription-based services to businesses. These platforms provide companies with access to sophisticated AI tools without the need for significant upfront investment in infrastructure or development. The SaaS model allows businesses to integrate AI into their operations seamlessly, solving complex challenges such as data analysis, predictive modeling, and automation without requiring deep technical expertise on their part.
For AI startups, this approach provides a consistent revenue stream while addressing the growing demand for AI-powered solutions across various industries. SaaS platforms cater to business needs by offering flexible subscription plans, customizable features, and the ability to scale up or down based on usage. The monetization strategy is often built around subscription tiers, value-added services, and performance-based pricing.
Key Benefits of B2B AI SaaS Platforms
- Scalability: AI solutions can be tailored to meet the needs of different business sizes and sectors.
- Cost-effectiveness: Clients avoid the upfront costs of software development, data infrastructure, and ongoing maintenance.
- Flexibility: Subscription models allow businesses to adjust their usage based on current requirements and growth.
Common Monetization Strategies
- Tiered Pricing: Different pricing models based on features, data usage, or access to advanced AI capabilities.
- Usage-Based Pricing: Charges based on the volume of data processed or the number of AI-driven tasks completed.
- Freemium Model: Offering basic functionality for free, while premium features and capabilities are available at a cost.
"AI startups that provide SaaS solutions often use a subscription-based model, offering a scalable way to tap into growing enterprise demand for AI tools."
Example Monetization Breakdown
Plan | Price | Features |
---|---|---|
Basic | $50/month | Limited access to core features, basic analytics |
Pro | $200/month | Advanced analytics, predictive tools, priority support |
Enterprise | $500/month | Custom AI solutions, unlimited usage, dedicated support |
Creating AI-Driven Products for Consumer Markets
AI startups can tap into consumer applications by developing innovative products that solve everyday problems. These products leverage AI's capabilities to offer smarter, more personalized experiences that traditional solutions cannot match. Consumer-focused AI tools range from virtual assistants to intelligent fitness apps, each designed to cater to specific user needs with advanced data analysis and automation.
The key to success in building consumer AI products lies in their ability to deliver value, whether through convenience, personalization, or improved decision-making. These products can be monetized through direct sales, subscription models, or even freemium offers. As AI continues to evolve, the demand for such solutions is expected to grow exponentially.
Approaches for Building AI-Powered Consumer Products
- Consumer-Centric Design: Focus on user needs and behaviors to create intuitive interfaces and seamless experiences.
- Data-Driven Personalization: Use AI to collect and analyze user data for tailored recommendations and content.
- Continuous Improvement: AI models should adapt and improve over time based on user interactions and feedback.
Revenue Streams for Consumer AI Products
- Subscription Model: Offer access to premium features or exclusive content through recurring payments.
- One-Time Purchases: Sell the AI product as a one-off purchase with lifetime access.
- Freemium: Provide a free version with limited features, and charge for advanced functionalities.
Examples of Consumer AI Products
Product | Description | Monetization |
---|---|---|
Smart Home Assistants | AI-powered devices that control home automation through voice commands. | Subscription for premium services, or direct sales of hardware |
AI Fitness Apps | AI-driven fitness trackers and workout planners that adapt to user progress. | Freemium model with in-app purchases |
AI-Powered Virtual Shopping | AI tools that assist in making personalized shopping decisions online. | Affiliate commissions, subscription for advanced features |
AI-driven consumer products must be built with a deep understanding of user psychology and behavior. Without this focus, even the most powerful AI models will struggle to resonate with customers.
Leveraging Data Licensing as a Revenue Model for AI Startups
Data licensing has emerged as a viable revenue stream for AI startups, particularly those that focus on developing specialized datasets or proprietary data processing algorithms. By offering access to unique data, AI startups can cater to industries ranging from healthcare to finance, where data-driven insights are crucial for innovation and operational efficiency. This model allows startups to monetize their data without necessarily building end-user products, creating a win-win situation for both the startup and its clients.
To successfully implement data licensing, AI companies typically need to establish a clear framework for pricing, data usage rights, and access levels. Often, startups license data in different tiers, providing more granular datasets to customers who require specific insights. The revenue generated through this approach is scalable, as more companies seek access to quality data for AI model training, business intelligence, or research purposes.
Key Benefits of Data Licensing for AI Startups
- Monetization of proprietary data: AI startups can transform valuable datasets into a direct source of revenue, instead of relying solely on product sales.
- Scalability: Licensing models allow startups to grow their revenue base without a proportional increase in operational costs.
- Market expansion: Data licensing opens up opportunities to serve multiple industries, broadening the startup's customer base.
Challenges and Considerations
It is essential for AI startups to ensure that they have the necessary infrastructure to handle data security, privacy, and compliance issues. Licensing data without addressing these concerns can lead to legal risks and reputational damage.
Types of Data Licensing Models
- Exclusive Licenses: This model gives clients exclusive access to a dataset for a certain period, typically at a premium price.
- Non-Exclusive Licenses: Clients share access to the data with others, making this a lower-cost option.
- Subscription-Based Licensing: Customers pay a recurring fee for continuous access to data, ensuring steady revenue for the startup.
Revenue Model Breakdown
License Type | Revenue Potential | Customer Base |
---|---|---|
Exclusive | High | Limited |
Non-Exclusive | Moderate | Large |
Subscription | Steady | Varied |
Exploring Subscription-Based Models for AI Tools and Services
Subscription-based models have become a common revenue stream for AI startups, offering flexibility for customers while providing predictable income for businesses. These models enable companies to provide AI-powered tools and services on a recurring basis, with varying pricing tiers tailored to the needs of different customer segments. By adopting subscription plans, AI startups can foster long-term relationships with their clients, ensuring consistent usage and providing opportunities for continuous improvement of their offerings.
There are multiple variations of subscription models that AI startups may adopt, ranging from freemium services to high-tier enterprise plans. These models often come with features that allow users to scale their subscriptions as their needs evolve. Below, we explore some key types of subscription plans that are popular among AI tool providers:
Key Subscription Models for AI Services
- Freemium Model: Provides a basic version of the AI tool for free, with premium features available through paid plans.
- Tiered Pricing: Offers different pricing tiers based on usage levels, such as number of users or amount of data processed.
- Pay-As-You-Go: Charges customers based on the actual consumption of the AI service, such as the number of API calls or processing power used.
- Enterprise Solutions: Custom pricing for large organizations with specific needs, often including premium support and tailored solutions.
"Subscription models not only provide AI startups with stable cash flow but also encourage continuous development and customer loyalty."
Comparing Subscription Plans
Plan Type | Price Range | Features |
---|---|---|
Freemium | Free with paid upgrades | Basic features, limited usage |
Tiered Pricing | $10–$100+/month | Multiple tiers, varying features |
Pay-As-You-Go | Variable | Usage-based pricing |
Enterprise | Custom | Custom solutions, premium support |
Strategic Collaborations with Major Corporations for AI Solutions
For AI startups, forming strategic alliances with established companies presents a lucrative opportunity to expand their market reach and enhance their technological capabilities. Large corporations often seek innovative AI solutions that can address specific challenges or streamline existing operations, creating a natural synergy for partnerships. In return, startups gain access to greater resources, industry expertise, and potential customer bases, which can accelerate growth and foster innovation.
These partnerships typically take the form of joint ventures, licensing agreements, or technology integration projects. By aligning with larger entities, AI startups can leverage the extensive networks and financial stability of their partners to scale their solutions more effectively. Additionally, these collaborations often include co-development of new products or services, allowing both parties to benefit from shared risks and rewards.
Key Benefits of AI Startups Partnering with Large Corporations
- Access to Resources: AI startups gain financial backing, industry knowledge, and a larger customer base.
- Increased Credibility: Collaboration with a well-established corporation enhances the startup’s reputation and trustworthiness in the market.
- Shared Innovation: Joint development allows both parties to bring their expertise to the table, resulting in superior products or services.
Types of Partnerships
- Joint Ventures: Both companies create a new, independent entity to co-develop AI technology and share the profits.
- Licensing Agreements: The startup licenses its AI technology to the large corporation for integration into their existing systems.
- Technology Integration: Startups integrate their AI solutions into the corporation’s products, providing both parties with a competitive edge.
Example Partnership Structure
Partner | Contribution | Benefit |
---|---|---|
AI Startup | Development of AI solutions and technologies | Access to a broader market and financial support |
Large Corporation | Funding, customer base, distribution channels | Innovative AI capabilities and competitive advantage |
"Strategic collaborations provide AI startups with the scalability and market presence they need, while large corporations benefit from cutting-edge innovations that drive their business forward."
AI as a Service: Providing Tailored AI Solutions to Industry-Specific Needs
AI startups are increasingly turning to a model that provides personalized AI solutions tailored to the unique needs of different sectors. This approach involves offering custom-built AI tools designed to solve specific problems within industries such as healthcare, finance, retail, and manufacturing. By understanding the distinct challenges faced by each sector, AI providers can develop algorithms and systems that deliver high-value insights and automation, ensuring their clients remain competitive in an ever-evolving market.
Unlike off-the-shelf AI products, these customized solutions require deep industry knowledge and the ability to integrate AI seamlessly into existing workflows. The service model revolves around building AI applications that fit precisely within the operational environment of a client, creating a high barrier to entry for competitors. This allows AI companies to charge premium fees for their tailored services, ensuring strong recurring revenue streams.
Benefits of AI Solutions for Specific Industries
- Increased Efficiency: Automation of repetitive tasks to save time and reduce errors.
- Enhanced Decision Making: Data-driven insights tailored to industry-specific challenges.
- Scalability: Solutions designed to grow alongside a company's operations and demands.
Industry Examples
- Healthcare: Custom AI solutions can help with patient diagnosis, predictive analytics, and personalized treatment plans.
- Retail: AI-driven inventory management systems, personalized shopping experiences, and demand forecasting.
- Finance: Risk assessment tools, fraud detection algorithms, and automated trading systems.
Key Considerations for AI Service Providers
Consideration | Explanation |
---|---|
Industry Expertise | Deep understanding of industry-specific problems and workflows is essential for developing valuable solutions. |
Customization | Tailoring AI solutions to the client’s operational needs enhances the relevance and effectiveness of the service. |
Integration | Seamless integration into existing systems is necessary to ensure smooth implementation and long-term value. |
AI as a service model thrives on offering deep, industry-specific insights, making it an indispensable tool for businesses looking to innovate and optimize their operations.
Generating Revenue from AI Consultancy and Advisory Services
AI consultancy and advisory services offer a lucrative revenue stream for AI startups by providing expert guidance and strategies to businesses looking to implement AI solutions. These startups leverage their deep technical knowledge and industry experience to help organizations understand and apply AI technologies effectively. By tailoring their offerings to meet the specific needs of various sectors, AI firms can attract clients from diverse industries, including healthcare, finance, and manufacturing.
Through these services, AI startups charge clients for strategic advice, technology recommendations, and system integration. Revenue generation is often driven by consulting fees, project-based contracts, and long-term advisory partnerships. The scalability of AI solutions and the growing demand for AI expertise further bolster the potential profitability of this business model.
Key Revenue Streams from AI Consultancy
- Project-Based Consulting: AI startups are hired to work on specific projects, providing bespoke solutions to solve business challenges.
- Strategy and Roadmap Development: Offering high-level strategic advice to help businesses align AI technologies with their goals.
- Implementation and Integration: Assisting companies in integrating AI into their existing infrastructure for seamless operations.
- Ongoing Support and Optimization: Providing continuous optimization and support for AI systems to ensure they evolve with changing business needs.
Monetization Models in AI Consultancy
- Hourly or Daily Rate: Charging based on the amount of time spent on a client's project.
- Fixed-Price Contracts: Pre-agreed flat rates for specific projects or consulting packages.
- Retainer Agreements: Long-term advisory services with a recurring monthly fee for continuous access to expertise.
- Success-Based Fees: Earning a fee tied to the successful implementation of AI systems that meet predefined performance metrics.
AI consultancy is not just about delivering solutions, but also about fostering long-term partnerships, where expertise becomes integral to the client’s ongoing success.
Examples of Revenue Models in AI Consultancy
Revenue Model | Description | Advantages |
---|---|---|
Hourly Consulting | Charges based on the number of hours spent on a client project. | Flexibility for both parties, ideal for short-term projects. |
Retainer Fees | Fixed monthly fee for ongoing access to consultancy services. | Stable, recurring revenue stream, fostering long-term relationships. |
Project-Based Pricing | Predefined price for a specific, clearly scoped project. | Clear expectations and deliverables, suitable for well-defined projects. |