Automation in Financial Services
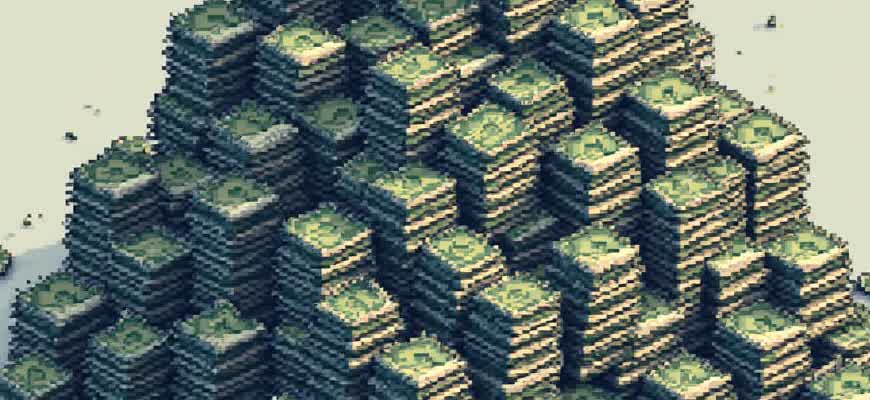
The integration of technology into financial services has transformed how institutions manage operations, process transactions, and interact with customers. By leveraging automation tools, businesses can achieve higher efficiency, reduce human error, and streamline routine tasks. This evolution is particularly evident in sectors such as banking, insurance, and investment management.
One of the key benefits of automation is the reduction in operational costs. Tasks that traditionally required extensive manual input, such as data entry or transaction processing, can now be performed by intelligent systems. These systems use algorithms to execute transactions with high accuracy and speed.
- Cost reduction
- Improved accuracy
- Faster service delivery
- Enhanced compliance tracking
"Automation in finance isn't just about cutting costs. It's about redefining how institutions provide services to customers and enhancing overall service quality."
In practice, automation has manifested in various forms:
- Automated trading systems
- Chatbots for customer support
- Robo-advisors for personalized investment strategies
Table 1 below shows a comparison of manual vs automated processes in financial services:
Process | Manual Process | Automated Process |
---|---|---|
Transaction Processing | Manual entry, time-consuming | Instant processing with algorithms |
Customer Support | Human agents, slow response | AI-driven chatbots, immediate response |
Compliance Checks | Periodic audits | Continuous real-time monitoring |
Optimizing Transaction Processing with Robotic Process Automation (RPA)
Robotic Process Automation (RPA) is transforming transaction processing within the financial services industry by automating repetitive tasks, improving accuracy, and reducing operational costs. With the ever-growing volume of financial transactions, companies are leveraging RPA to streamline workflows, enhance productivity, and ensure faster processing times. By integrating RPA into transaction management, financial institutions can minimize manual intervention and optimize the efficiency of complex tasks such as data entry, reconciliation, and reporting.
RPA systems are capable of handling high-volume transactions with minimal error rates. These intelligent bots can interact with multiple applications, extract necessary data, and execute predefined steps without human oversight. As a result, financial organizations can maintain compliance, reduce risks associated with human error, and improve customer satisfaction through quicker transaction processing.
Key Benefits of RPA in Transaction Processing
- Increased Efficiency: RPA reduces the time required for transaction processing by automating repetitive tasks.
- Cost Reduction: Automation helps lower operational costs by minimizing the need for manual labor.
- Improved Accuracy: Bots eliminate the risk of human error, ensuring data consistency and reliability.
- Scalability: RPA solutions can handle a higher volume of transactions, making them ideal for growth periods.
Implementation Process
- Identifying Suitable Tasks: Analyze current workflows to determine which tasks are best suited for automation, such as invoice processing or payment matching.
- Bot Development: Design and deploy automation bots with the ability to perform specific tasks efficiently.
- Integration: Ensure RPA tools are integrated with existing systems for seamless data exchange and task execution.
- Monitoring and Optimization: Continuously monitor bot performance and fine-tune processes for optimal results.
Impact on Business Operations
By automating transaction processing, financial institutions not only increase operational efficiency but also create a more secure environment for their clients. RPA helps businesses stay competitive by maintaining high service standards and delivering faster transaction turnaround times.
Sample Transaction Processing Workflow
Step | Action | Responsible Party |
---|---|---|
1 | Transaction Data Collection | RPA Bot |
2 | Data Verification | RPA Bot |
3 | Payment Processing | RPA Bot |
4 | Transaction Confirmation | RPA Bot |
How AI-Powered Systems Enhance Fraud Detection in Real-Time
Artificial Intelligence (AI) has transformed fraud detection in financial services by enabling systems to identify suspicious activities in real-time. Traditional methods were largely based on rule-based algorithms and manual reviews, which were time-consuming and often ineffective in fast-evolving fraud scenarios. AI, however, can analyze large volumes of transaction data instantly, recognizing patterns and anomalies that would be impossible for human analysts to catch in time.
AI-powered systems utilize machine learning models that adapt and improve their detection capabilities as they process more data. By continuously learning from new fraud tactics, these systems provide more accurate and timely alerts, preventing financial losses and enhancing customer trust. The use of AI in fraud prevention not only boosts the speed of detection but also reduces false positives, ensuring that legitimate transactions are not delayed.
How AI Works in Real-Time Fraud Detection
- Data Analysis: AI systems analyze transaction data, including user behavior, transaction amount, and frequency, to identify patterns indicative of fraud.
- Behavioral Profiling: Machine learning models create a baseline of normal user behavior, enabling the system to detect deviations that suggest fraudulent activity.
- Real-Time Alerts: As soon as the system detects a potential fraud pattern, it generates an alert, allowing immediate action to be taken.
Key Benefits of AI in Fraud Detection
- Speed: AI models process and analyze transactions faster than human investigators, minimizing the risk of undetected fraud.
- Accuracy: By continually learning from past fraud cases, AI reduces the likelihood of false positives, ensuring that legitimate transactions go through smoothly.
- Scalability: AI systems can handle a vast amount of transactions simultaneously, making them suitable for large-scale financial institutions.
"AI-powered fraud detection systems can identify complex fraud schemes in real time, providing financial institutions with the ability to act instantly and prevent losses."
Example of AI in Fraud Detection
Method | Description |
---|---|
Transaction Monitoring | AI systems analyze each transaction to detect abnormal behavior or patterns, such as large, rapid transfers. |
Geolocation Tracking | AI compares the geographic location of transactions to typical user locations, flagging anomalies like purchases made far from the user's usual area. |
Device Recognition | AI identifies whether a transaction is being made from a device previously used by the customer, helping to spot unauthorized access. |
Automating Customer Onboarding: Streamlining Compliance and Risk Assessment
In the financial services industry, the customer onboarding process is a critical step, involving the collection of sensitive information and the assessment of potential risks. Automating this process can significantly reduce the time and effort required to onboard new clients while ensuring compliance with regulatory requirements. By leveraging technology, financial institutions can minimize human error and enhance operational efficiency.
Automation tools can streamline various aspects of the onboarding process, from identity verification to risk assessments. These tools can instantly verify the authenticity of documents and assess the customer’s risk profile based on a set of pre-determined criteria. As a result, banks and financial services providers can improve customer experience while reducing the burden of manual checks and compliance-related tasks.
Key Advantages of Automating Onboarding
- Enhanced Compliance: Automated systems ensure that financial institutions adhere to regulatory guidelines without missing important steps in the verification process.
- Improved Efficiency: Automation reduces the time spent on manual tasks, allowing staff to focus on more value-added activities.
- Accurate Risk Profiling: Algorithms can instantly evaluate customer risk levels by cross-referencing various data points and flagging high-risk customers.
Compliance and Risk Assessment Automation Flow
- Identity Verification: Automated tools cross-check personal details with government databases to confirm identity.
- Document Verification: AI scans and verifies submitted documents for authenticity and completeness.
- Risk Profiling: Analyzing financial history, transaction behavior, and geolocation to assess potential risks.
- Regulatory Compliance Checks: Automated systems cross-reference customer data with blacklists, sanction lists, and other regulatory sources.
"By automating the onboarding process, financial institutions not only speed up the workflow but also reduce the likelihood of human error, ensuring more accurate compliance with regulatory standards."
Comparison of Manual vs Automated Onboarding
Aspect | Manual Onboarding | Automated Onboarding |
---|---|---|
Time Consumption | High | Low |
Compliance Risk | High | Low |
Accuracy | Moderate | High |
Customer Experience | Slow | Fast |
Reducing Operational Costs Through Automated Data Entry and Reporting
Financial institutions often deal with vast amounts of data that need to be accurately entered, processed, and reported regularly. Manual data entry can be error-prone and time-consuming, significantly increasing operational expenses. Automation in data entry and reporting addresses these challenges by streamlining repetitive tasks, reducing human intervention, and minimizing the risk of mistakes. This not only improves the efficiency of operations but also leads to substantial cost savings for financial organizations.
By integrating automated systems, financial services can eliminate the need for extensive manual labor while enhancing data accuracy and processing speed. Automation tools can also optimize reporting, allowing real-time access to crucial financial data without delays. The reduction in human involvement and paper-based workflows directly contributes to a leaner, more cost-efficient operation.
Key Benefits of Automation in Data Entry and Reporting
- Cost Reduction: Automated systems minimize the need for large manual workforce, reducing labor and training costs.
- Improved Accuracy: Automation reduces human error, ensuring precise data entry and reliable reporting.
- Faster Processing: Tasks that once took hours can now be completed in minutes, speeding up decision-making processes.
- Scalability: Automated systems can handle increasing data volumes without additional resource requirements.
Impact on Reporting Efficiency
Financial reporting typically involves large datasets that must be compiled and analyzed. Automated tools can generate comprehensive reports in real-time, allowing businesses to respond more quickly to market changes.
"Automation in financial reporting significantly reduces the time spent on manual data compilation, leading to faster insights and better-informed decisions."
Process | Manual | Automated |
---|---|---|
Data Entry | Hours of work | Minutes of processing |
Error Rate | High | Minimal |
Report Generation | Days | Real-time |
Conclusion
Automated data entry and reporting not only streamline operations but also deliver long-term savings by reducing labor costs, enhancing accuracy, and speeding up processes. The financial industry can leverage these technologies to maintain competitive advantage and better serve their clients.
Enhancing Investment Management with Algorithmic Trading Solutions
Investment management has seen a remarkable transformation with the advent of algorithmic trading. By utilizing advanced mathematical models and automated systems, financial institutions can execute complex trading strategies at scale, faster, and with more precision than ever before. These systems are designed to process vast amounts of data in real time, optimizing investment decisions and reducing human error, which has traditionally plagued manual trading approaches.
Algorithmic trading allows for the automation of trade execution, ensuring consistent strategies and reducing the emotional bias often present in human decision-making. With algorithms in place, firms can also leverage market inefficiencies and capitalize on arbitrage opportunities that might otherwise go unnoticed. The increased accuracy and speed of execution contribute significantly to enhancing overall portfolio performance and minimizing risks.
Key Benefits of Algorithmic Trading
- Increased Speed: Automated systems can execute trades in milliseconds, far outpacing human traders and capitalizing on short-term market fluctuations.
- Cost Efficiency: With reduced need for human intervention, firms can lower operational costs while improving trade execution quality.
- Data-Driven Decisions: Algorithms analyze vast datasets to identify trends, patterns, and anomalies that would be difficult for a human to process in real-time.
- Minimized Market Impact: Algorithms can break large orders into smaller ones to avoid significant price impacts, ensuring smoother transactions.
Implementation Process in Investment Management
- Data Collection: Gather historical and real-time market data to feed the algorithm and define strategies.
- Model Development: Design mathematical models based on statistical and machine learning techniques that align with the firm’s trading goals.
- Backtesting: Test the algorithm’s performance using historical data to evaluate effectiveness before live deployment.
- Execution: Deploy the algorithm for real-time trading, ensuring continuous monitoring and optimization of performance.
"Algorithmic trading has revolutionized how investment firms manage portfolios, ensuring optimal execution and risk management at unprecedented speeds."
Performance Comparison
Metric | Traditional Trading | Algorithmic Trading |
---|---|---|
Execution Speed | Slow (manual execution) | Milliseconds (automated execution) |
Cost Efficiency | Higher operational costs | Lower due to automation |
Risk Management | Susceptible to emotional bias | Based on data-driven algorithms, reducing human error |
Integrating Blockchain with Automation for Secure Financial Transactions
In the ever-evolving landscape of financial services, ensuring the security and efficiency of transactions is crucial. The combination of blockchain technology and automation offers a powerful solution to address these challenges. Blockchain provides a decentralized ledger, ensuring transparency and immutability, while automation enhances the speed and accuracy of transactions. Together, they create a robust framework for securely processing financial operations in real time.
By integrating these technologies, financial institutions can streamline processes, reduce human error, and eliminate the need for intermediaries. This synergy results in faster transaction times, lower costs, and higher security standards. Here’s how blockchain and automation can work in harmony to strengthen financial transactions:
Key Benefits of Blockchain and Automation Integration
- Enhanced Security: Blockchain’s decentralized nature ensures that data is tamper-resistant, while automated systems remove the human element, reducing the risk of fraud and errors.
- Improved Efficiency: Automation speeds up transaction processes, while blockchain guarantees that each step is validated and recorded securely, eliminating delays.
- Cost Reduction: By eliminating intermediaries and automating tasks like compliance checks and data validation, organizations can reduce operational costs.
How it Works: The Automation-Blockchain Connection
- Transaction Initiation: The process begins when a financial request is made, which triggers an automated smart contract to verify the details.
- Smart Contract Validation: A smart contract, based on blockchain technology, automatically checks the requirements and executes the transaction if all conditions are met.
- Data Recording: Every transaction is recorded on the blockchain, creating an immutable, transparent record that cannot be altered.
"The integration of blockchain and automation offers unprecedented levels of security, transparency, and efficiency for financial transactions, ensuring trust in every step of the process."
Real-World Application: Blockchain in Payment Systems
Feature | Blockchain-Enabled Automation |
---|---|
Transaction Time | Instantaneous, without the need for third-party validation. |
Security | Encrypted and immutable transaction records. |
Cost Efficiency | Reduction in fees by eliminating intermediaries and manual processes. |
Improving Loan Processing Efficiency with Machine Learning
Loan approval processes in financial institutions can often be slow and cumbersome, causing delays for both lenders and borrowers. Traditional methods of evaluating creditworthiness rely heavily on manual data entry and rule-based systems, which can lead to bottlenecks and human error. In contrast, machine learning (ML) models offer a more streamlined and automated approach to loan decision-making, allowing for quicker and more accurate approvals.
By leveraging advanced ML techniques, financial institutions can significantly reduce the time it takes to assess loan applications. These models analyze large datasets, identify patterns, and make predictions based on a borrower’s financial history, behavior, and other relevant factors. This automation not only speeds up the approval process but also enhances the precision of the decisions made.
How Machine Learning Optimizes Loan Approval
- Data Analysis: ML algorithms can quickly process vast amounts of data, including credit scores, transaction history, and even non-traditional data sources such as social media activity.
- Automated Decision-Making: Machine learning models can make real-time, data-driven decisions, reducing the need for manual intervention and improving consistency in loan approvals.
- Risk Assessment: ML models can more accurately predict a borrower’s risk level by considering a wider range of factors, thus allowing lenders to offer more tailored loan products.
Key Benefits of Using Machine Learning in Loan Approval
- Faster Processing: ML models can analyze and approve loan applications in a fraction of the time compared to traditional methods.
- Reduced Bias: By relying on data-driven models, lenders can minimize human bias, ensuring that decisions are made based solely on objective criteria.
- Enhanced Customer Experience: Borrowers benefit from quicker responses and more personalized loan offers.
Example of Machine Learning in Action
ML Model Type | Function | Impact on Loan Processing |
---|---|---|
Logistic Regression | Predicts loan approval likelihood based on applicant data | Faster initial screening and reduced time to decision |
Random Forest | Analyzes multiple factors to classify risk levels | Improved accuracy in risk assessment |
Neural Networks | Detects complex patterns in borrower behavior | More precise loan terms and conditions |
"Machine learning transforms the traditional loan approval process by enabling faster, more accurate, and bias-free decision-making, ultimately benefiting both lenders and borrowers."