Potential Ai Companies
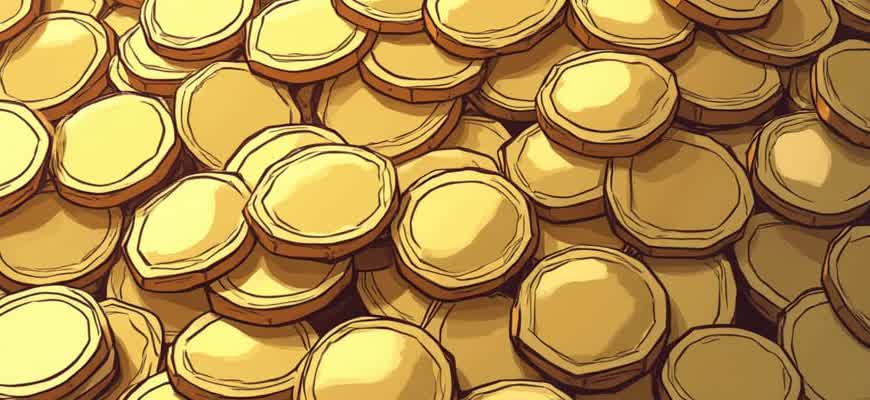
The AI industry is rapidly evolving, with many startups and established companies leading the charge in developing cutting-edge technologies. These businesses are pushing the boundaries of what artificial intelligence can accomplish, ranging from machine learning to natural language processing, and autonomous systems. Below are some of the key areas where AI companies are making significant strides.
- Healthcare: AI-driven diagnostics, personalized treatment plans, and robotic surgery.
- Finance: Algorithms for predictive analytics, fraud detection, and automated trading systems.
- Autonomous Vehicles: Self-driving technologies and AI-based traffic management systems.
These industries, along with several others, represent the driving force behind the rise of AI startups and their disruptive potential. Below is a table outlining some of the key companies and their focus areas:
Company | Industry | Focus Area |
---|---|---|
OpenAI | Technology | Natural language processing and AI research |
DeepMind | Healthcare | AI for medical research and treatment |
Waymo | Automotive | Self-driving cars |
"The next generation of AI companies will shape the future of how we interact with technology, solve complex problems, and improve industries worldwide."
How to Select the Right AI Partner for Your Business
Choosing the right artificial intelligence company is crucial for the success of your business’s AI strategy. With the wide range of options available, it’s essential to focus on a company that aligns with your business goals and can scale with your needs. Here’s how to make an informed decision.
The process of finding the right AI company involves assessing key factors such as expertise, previous experience, and technological compatibility. It is also vital to understand how well the company can tailor their solutions to solve your specific challenges. To help guide you through this process, consider the following criteria.
Key Factors to Consider
- Experience and Track Record: Check the company’s history with projects similar to yours. Look for case studies, client testimonials, or any published results.
- Technology Compatibility: Ensure the AI solutions offered are compatible with your existing systems and can be seamlessly integrated.
- Scalability: The AI solution must grow with your business. Assess if the company can adjust the technology as your needs evolve.
- Support and Training: A good AI partner should provide ongoing support and training to your team to maximize the solution’s potential.
Questions to Ask Before Making a Decision
- What specific AI tools and frameworks do you use? Make sure the company’s technology stack fits your business requirements.
- Can you provide examples of your work with businesses of our size? Understanding their experience with similar-scale projects is crucial.
- What is your approach to data security and privacy? This ensures that sensitive data will be handled appropriately and securely.
- What are the long-term costs of implementation and maintenance? Consider the total cost of ownership, not just initial development expenses.
Important Considerations
Investing in AI is a long-term commitment. Choosing the right partner is not just about technology, but about aligning with a company that understands your vision and can adapt their solutions over time.
Comparison Table
Criteria | Company A | Company B | Company C |
---|---|---|---|
Experience | 5+ years in retail AI solutions | Expert in healthcare AI | General AI solutions for SMBs |
Technology Compatibility | Compatible with most ERP systems | Custom AI for medical records | Cloud-based AI integration |
Scalability | High scalability for large retailers | Limited scalability for small practices | Highly scalable for growing businesses |
Support and Training | Comprehensive training & 24/7 support | Basic training and limited support | Extensive support with personalized training |
Key Factors to Consider When Evaluating AI Solutions
When assessing AI technologies for your business, it is essential to consider a range of factors that directly influence the effectiveness, scalability, and sustainability of the solution. These factors determine how well the AI integrates into existing systems, addresses business goals, and offers long-term value. A thorough evaluation goes beyond simply analyzing the technology itself and includes aspects related to data quality, system adaptability, and potential for future growth.
Moreover, understanding the specific needs of your organization and aligning them with the capabilities of the AI solution is crucial for successful implementation. Here are the primary considerations that can guide your decision-making process when selecting AI solutions.
1. Data Quality and Availability
Data is the cornerstone of any AI solution. The accuracy and volume of data you have will significantly affect the performance of the AI model. Evaluate the following:
- Data Source Reliability: Ensure your data is reliable, accurate, and comes from trustworthy sources.
- Data Quantity: Large datasets improve AI models, but consider the quality over sheer volume.
- Data Accessibility: The AI system should be able to access and process data from various sources seamlessly.
2. Model Transparency and Explainability
Understanding how an AI model makes decisions is crucial for trust and accountability. You need a solution that offers insight into its decision-making process:
- Interpretability: Choose AI systems that provide clear explanations of how outcomes are reached.
- Transparency: Transparency in AI allows for easier troubleshooting and optimization.
- Compliance with Regulations: Make sure the model complies with any industry-specific regulations regarding AI usage.
3. Integration with Existing Infrastructure
It is important to assess how well the AI solution will integrate with your current IT infrastructure and workflows. A seamless integration process reduces operational friction:
- System Compatibility: The AI solution should align with your current software and hardware setups.
- Scalability: The solution should be able to grow with your business without requiring significant reconfigurations.
- Ease of Use: Consider the learning curve for employees and the need for specialized training.
"Investing in AI without considering its adaptability to your existing systems is like trying to fit a square peg into a round hole."
4. Cost and ROI Potential
While the potential benefits of AI can be significant, it’s important to weigh these against the financial investment required:
Cost Factor | Consideration |
---|---|
Initial Implementation Cost | Assess the upfront costs for deployment and integration. |
Maintenance and Updates | Consider ongoing costs for system updates and maintenance. |
Long-Term Value | Evaluate the ROI in terms of improved efficiency, reduced costs, and enhanced decision-making capabilities. |
How AI Companies Tailor Solutions for Different Industries
AI companies create customized solutions to meet the unique demands of various sectors. By leveraging machine learning, data analytics, and automation technologies, these companies develop products that address industry-specific challenges and improve operational efficiency. For instance, in healthcare, AI-driven systems are used for diagnostic assistance, while in finance, algorithms optimize risk management and trading strategies. The key to success lies in the ability to adapt AI technologies to the distinct processes and regulatory frameworks of each industry.
Through a deep understanding of industry needs, AI companies implement solutions that enhance productivity, reduce costs, and provide valuable insights. Customization often involves collaboration between AI developers and industry experts to ensure that the technology aligns with specific workflows, goals, and compliance requirements. Below are examples of how AI is being applied across different sectors.
AI in Different Industries
- Healthcare: AI-powered diagnostic tools, predictive analytics for patient outcomes, and robotic surgery systems.
- Finance: Fraud detection, algorithmic trading, and risk assessment models.
- Retail: Personalized recommendations, demand forecasting, and supply chain optimization.
- Manufacturing: Predictive maintenance, quality control automation, and production line optimization.
- Automotive: Autonomous vehicles, predictive maintenance, and supply chain efficiency.
Key Approaches for Tailoring AI Solutions
- Industry-specific data analysis: Gathering and processing large datasets relevant to each sector helps AI companies create models that are fine-tuned for specific use cases.
- Scalable AI architectures: Developing solutions that can grow with industry demands, ensuring flexibility and long-term viability.
- Collaborative development: Engaging with industry professionals to create bespoke AI systems that understand the intricacies of the sector.
"AI solutions must be designed with the unique challenges of each industry in mind to achieve meaningful impact and deliver measurable results."
Example Comparison: Healthcare vs. Finance
Industry | AI Application | Purpose |
---|---|---|
Healthcare | AI-based diagnostic tools | Improving diagnostic accuracy and speeding up patient care |
Finance | Algorithmic trading | Optimizing market decisions and reducing human error in trades |
The Role of Custom AI Development in Business Transformation
In the modern business landscape, customized AI solutions have become essential for driving growth and innovation. Tailored artificial intelligence systems provide organizations with the ability to address specific operational challenges, enhance customer experiences, and streamline internal processes. Unlike off-the-shelf AI tools, custom development ensures that the solutions align closely with the company’s strategic goals, offering a competitive edge that cannot be easily replicated.
Implementing custom AI technology requires careful consideration of a company’s unique needs and industry dynamics. By integrating AI systems that are specifically designed to handle industry-specific tasks, businesses can optimize their workflows, improve decision-making, and reduce costs. This approach also fosters long-term scalability, allowing companies to adjust as their requirements evolve.
Key Benefits of Custom AI for Businesses
- Efficiency Enhancement: AI systems can automate repetitive tasks, freeing up human resources for more strategic initiatives.
- Personalized Customer Experiences: AI can analyze customer data to deliver highly relevant and customized recommendations, improving satisfaction and loyalty.
- Data-Driven Decisions: Custom AI can process and analyze vast amounts of data quickly, providing actionable insights to improve business strategies.
"Custom AI development allows businesses to not just adopt technology but to tailor it to their specific needs, ensuring maximum impact on performance and growth."
Steps for Implementing Custom AI Solutions
- Identify Key Objectives: Clearly define the business goals that AI can address, such as automation, customer service, or predictive analytics.
- Data Collection and Preparation: Gather and clean relevant data to train AI models effectively, ensuring high-quality insights.
- Develop and Integrate: Work with AI experts to build and integrate the custom solution into existing systems.
- Continuous Improvement: Monitor performance and refine AI models regularly to adapt to changing business needs.
Comparison: Custom vs. Off-the-Shelf AI
Aspect | Custom AI | Off-the-Shelf AI |
---|---|---|
Adaptability | Highly tailored to specific needs | General solutions, limited flexibility |
Cost | Higher initial investment, long-term ROI | Lower upfront cost, limited returns |
Scalability | Scalable based on business growth | Limited scalability options |
How to Evaluate the Return on Investment with AI Partners
Measuring the return on investment (ROI) when collaborating with AI solution providers is crucial for businesses aiming to assess the effectiveness of their AI strategies. Given the complexity and long-term nature of AI projects, traditional financial metrics might not be enough to capture all aspects of value. Instead, a combination of both quantitative and qualitative indicators is needed to determine how AI technologies contribute to overall business success.
One of the main challenges in evaluating ROI is identifying the appropriate metrics that truly reflect the impact of AI. These indicators can vary depending on the AI solution’s scope, industry application, and specific business goals. Below are several key factors to consider when assessing ROI in AI partnerships.
Key Metrics for Measuring ROI
- Operational Efficiency Gains: Measure improvements in productivity, cost reductions, and resource optimization.
- Customer Satisfaction: Evaluate the AI's influence on customer service, engagement, and retention through improved personalized experiences.
- Time to Market: Assess how AI accelerates product development and reduces time for new features or solutions to reach the market.
- Revenue Growth: Examine the direct or indirect revenue increase attributed to AI-driven decisions, new offerings, or business model innovations.
Evaluating Financial Impact
To translate these metrics into a clear financial picture, businesses can follow a structured approach:
- Cost-Benefit Analysis: Compare the total cost of AI implementation, including hardware, software, and human resources, with the tangible benefits over a defined period.
- Value Quantification: Assign monetary values to operational efficiencies, time savings, and revenue enhancements to determine the overall financial impact.
- Break-even Analysis: Calculate how long it will take for the AI investment to pay off, considering both short-term and long-term returns.
Example of ROI Calculation
Metric | Value |
---|---|
Initial Investment | $500,000 |
Operational Savings | $200,000/year |
Additional Revenue | $100,000/year |
ROI (3 years) | ($200,000 + $100,000) * 3 - $500,000 = $100,000 |
Important: ROI calculation should not only focus on direct financial returns but also consider factors like improved brand reputation and market positioning due to AI-driven innovation.
Common Pitfalls to Avoid When Selecting an AI Partner
When selecting a partner to develop or implement AI solutions, it is critical to ensure that the organization aligns with your business goals and technical requirements. Many companies overlook the complexity of AI projects, which can lead to delays, wasted resources, and unmet expectations. Understanding the potential risks in this process can help mitigate future problems.
Choosing the wrong AI partner can be costly in both time and investment. There are specific red flags that can indicate an unsuitable fit, and being aware of these issues can save your company from future headaches. Below are some common pitfalls to avoid when selecting an AI partner for your business.
1. Lack of Clear Communication and Understanding
It is essential to establish clear communication from the outset. Misunderstandings about project scope, timelines, and expectations can derail the entire process. Ensure that both parties understand the goals, technical capabilities, and limitations of the AI solution.
- Ensure transparent communication channels
- Establish clear goals and KPIs
- Discuss potential risks upfront
2. Overpromising Capabilities
Some AI companies may present overly optimistic claims about their capabilities, which can lead to disappointment if those promises are not met. Always verify the partner's expertise, review case studies, and ask for tangible evidence of previous work.
Key tip: Avoid companies that promise solutions without providing realistic timelines or benchmarks.
3. Inadequate Post-Implementation Support
AI systems require ongoing maintenance and refinement to remain effective. A common oversight is failing to assess the level of post-implementation support a partner offers. Without continuous support, your AI solution may become outdated or fail to perform as intended.
- Ask about long-term support plans
- Evaluate the partner’s approach to system updates
- Ensure they offer troubleshooting services post-launch
4. Misalignment of Business and AI Objectives
It's crucial that the AI strategy aligns with your broader business objectives. A partner that focuses only on technology without understanding your industry-specific needs may deliver solutions that don’t fully address your challenges.
Common Misalignments | Consequences |
---|---|
Focusing on technical features over business outcomes | Poor return on investment, missed business opportunities |
Developing one-size-fits-all solutions | Lack of scalability or customization, inefficiency |
Understanding the Implementation Timeline for AI Solutions
Integrating artificial intelligence into business processes can vary significantly in terms of time and complexity. The timeline is highly dependent on the scope of the AI application, the infrastructure in place, and the specific needs of the organization. For companies planning to implement AI, it’s crucial to establish a clear and realistic timeline that aligns with both short-term goals and long-term strategic objectives.
Several factors influence how long it will take to implement AI solutions, including data availability, required model training, and system integration. The process typically unfolds in distinct phases, with each requiring careful planning and attention. Below, we outline the general stages and typical duration of implementing AI technologies in a business setting.
Phases of AI Implementation
- Needs Assessment and Goal Setting: In this phase, businesses define the AI's purpose, such as automating tasks or improving decision-making processes. This stage typically lasts from 2 to 4 weeks.
- Data Collection and Preparation: Gathering and preparing high-quality data is crucial for training AI models. This can take anywhere from 1 to 3 months, depending on the complexity of the data.
- Model Development and Training: Developing AI models requires significant computational resources. This stage can range from 2 to 6 months, depending on the sophistication of the solution.
- Testing and Refinement: After the model is developed, it undergoes testing and fine-tuning to ensure accuracy and reliability. This phase might take 1 to 2 months.
- Deployment and Monitoring: The final step is rolling out the solution into production, followed by continuous monitoring. This can span from a few weeks to several months, based on the scale of the deployment.
Critical Time Considerations
It’s important to remember that the timeline can fluctuate based on unforeseen challenges such as data quality issues or integration difficulties. Flexibility is key to a successful AI deployment.
Example of AI Project Timeline
Phase | Duration |
---|---|
Needs Assessment | 2-4 weeks |
Data Preparation | 1-3 months |
Model Development | 2-6 months |
Testing | 1-2 months |
Deployment | 2-4 months |